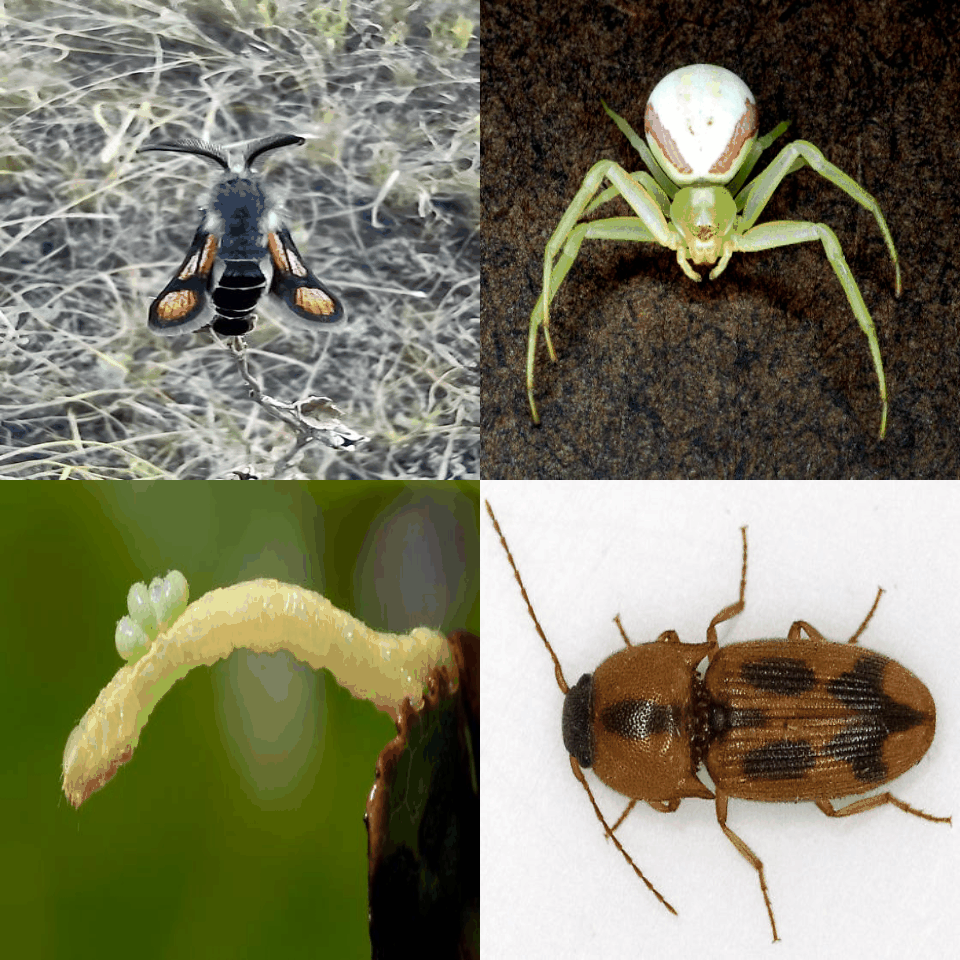
Insect Foundation Model
Insect-related disasters are one of the most important factors affecting crop yield due to the fast reproduction and widely distributed, large variety of insects. In the agricultural revolution, detecting and recognizing insects plays an important role in the ability for crops to grow healthily and produce a high-quality yield. To achieve this, insect recognition helps to differentiate between bugs that must be targeted for pest control and bugs that are essential for protecting farms. Machine learning, especially deep learning requires a large volume of data to achieve high performance. Therefore, we introduce a novel "Insect Foundation" dataset, a game-changing resource poised to revolutionize insect-related foundation model training. This rich and expansive dataset consists of 900,000 images with dense labels of taxonomy hierarchy from the high level of taxonomy (e.g., Class, Order) to the low level of taxonomy (e.g., Genus, Species). Covering a vast spectrum of insect species, our dataset offers a panoramic view of entomology, enabling foundation models to comprehend visual and semantic information about insects like never before. Our proposed dataset carries immense value, fostering breakthroughs across precision agriculture and entomology research. Insect Foundation Dataset promises to empower the next generation of insect-related AI models, bringing them closer to the ultimate goal of precision agriculture.
Link
Link
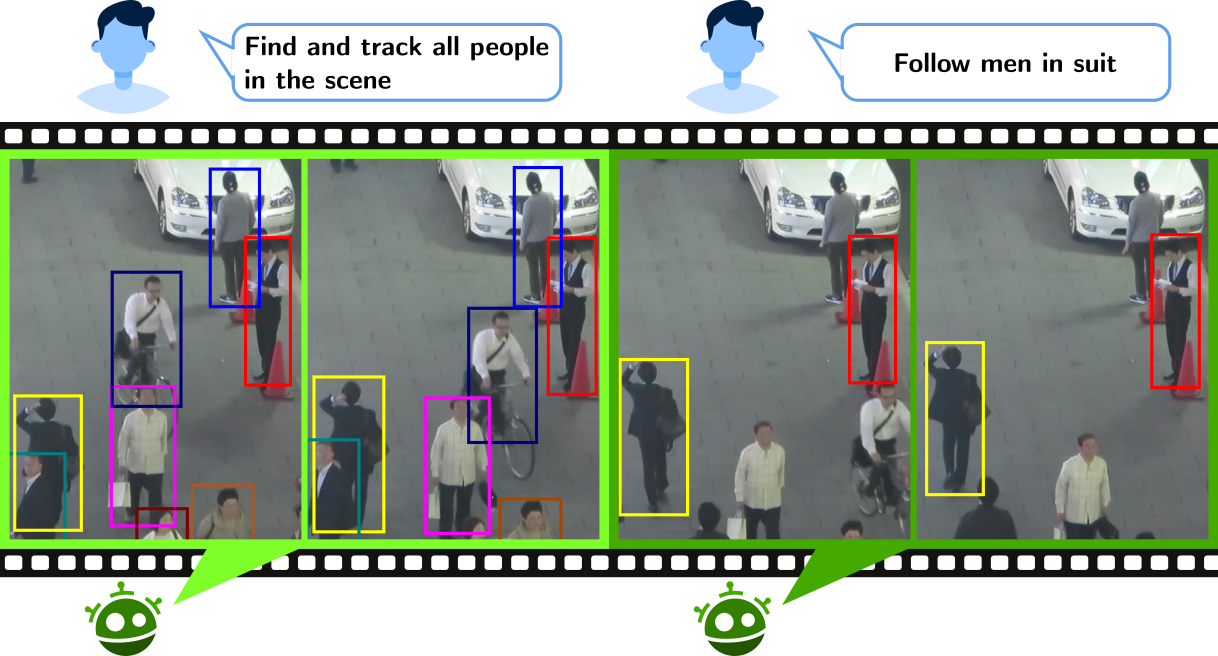
Type-to-Track: Retrieve Any Object via Prompt-based Tracking
One of the recent trends in vision problems is to use natural language captions to describe the objects of interest. This approach can overcome some limitations of traditional methods that rely on bounding boxes or category annotations. This paper introduces a novel paradigm for Multiple Object Tracking called Type-to-Track, which allows users to track objects in videos by typing natural language descriptions. We present a new dataset for that Grounded Multiple Object Tracking task, called GroOT, that contains videos with various types of objects and their corresponding textual captions describing their appearance and action in detail. Additionally, we introduce two new evaluation protocols and formulate evaluation metrics specifically for this task. We develop a new efficient method that models a transformer-based eMbed-ENcoDE-extRact framework (MENDER) using the third-order tensor decomposition. The experiments in five scenarios show that our MENDER approach outperforms another two-stage design in terms of accuracy and efficiency, up to 14.7% accuracy and 4× speed faster.
Link
Link
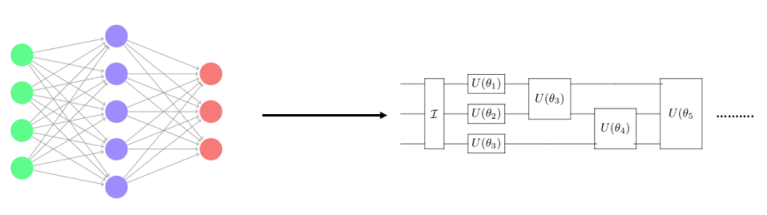
Quantum Machine Learning and Autonomous 2D Crystals Identification
Classical neural network algorithms are computationally expensive. For example, in image classification, representing an image pixel by pixel using classical information requires an enormous amount of computational memory resources. Hence, exploring methods to represent images in a different paradigm of information is important. We proposed a parameter encoding scheme for defining and training neural networks in quantum information based on time evolution of quantum spaces.
Link
Link
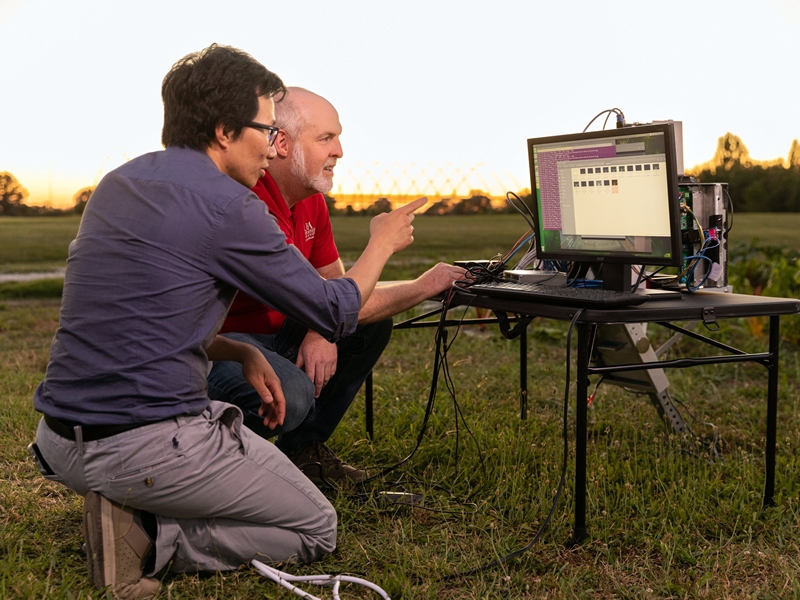
Researchers Receive NSF Funding to Continue Building a Smarter Insect Trap
Ashley Dowling, a researcher in the U of A System Division of Agriculture and professor of entomology and plant pathology, and Khoa Luu, an assistant professor of computer science and computer engineering, are leading development of the smarter insect trap.
Link
Link
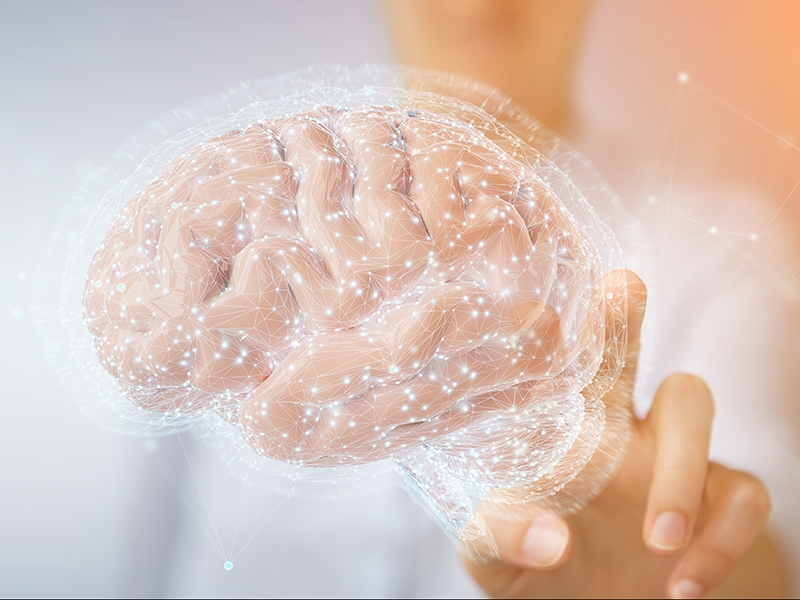
Graduate Students Take Third Place in MIT's Algonauts Project 2023 Challenge
Xuan Bac Nguyen, a Ph.D. candidate in the Department of Electrical Engineering and Computer Science, and his team placed third in the MIT Vision Brain Challenge, Algonauts Project 2023. The competition featuring more than 100 research teams around the world judges how successfully computational AI models predict brain responses to visual stimuli of natural scenes.
Link
Link
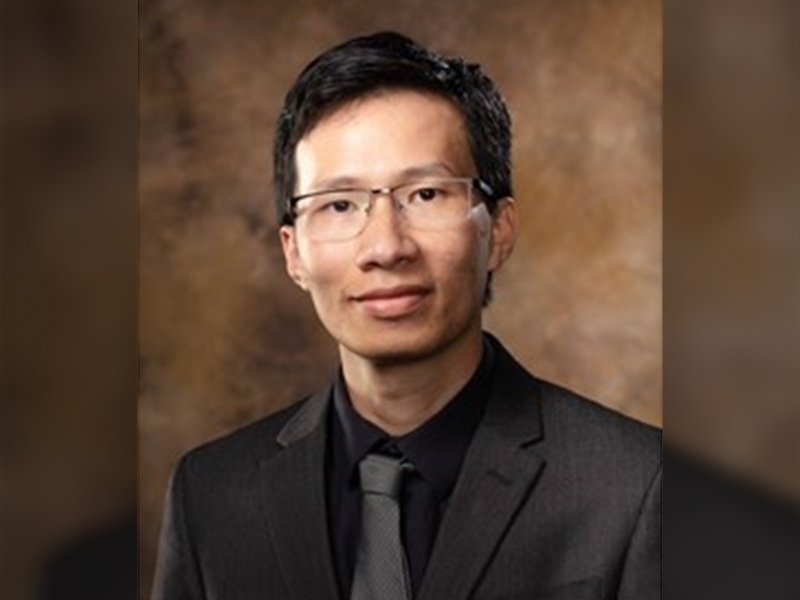
Technology Ventures Inventor Spotlight: Khoa Luu
Recently Dr. Luu has been working on the problem that manually managing insects seems impossible as the size of a farm gets larger. Therefore, the ability to automatically detect and identify insects is a primary demand in the area of crop management. Despite the many advances in precision agriculture, this is one area still largely reliant on manual labor. A primary objective in this area is the ability to identify and count insect species in real-time.
Link
Link
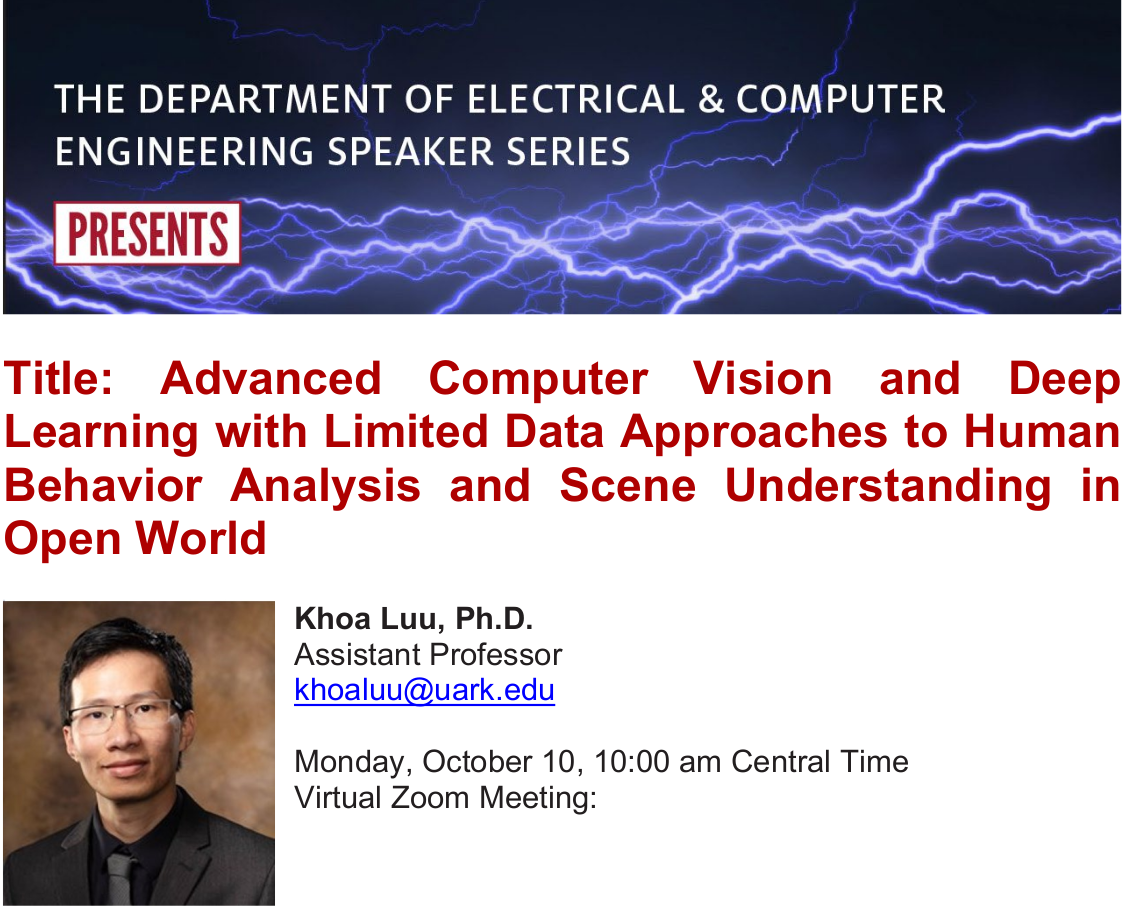
Advanced Computer Vision and Deep Learning with Limited Data Approaches to Human Behavior Analysis and Scene Understanding in Open World
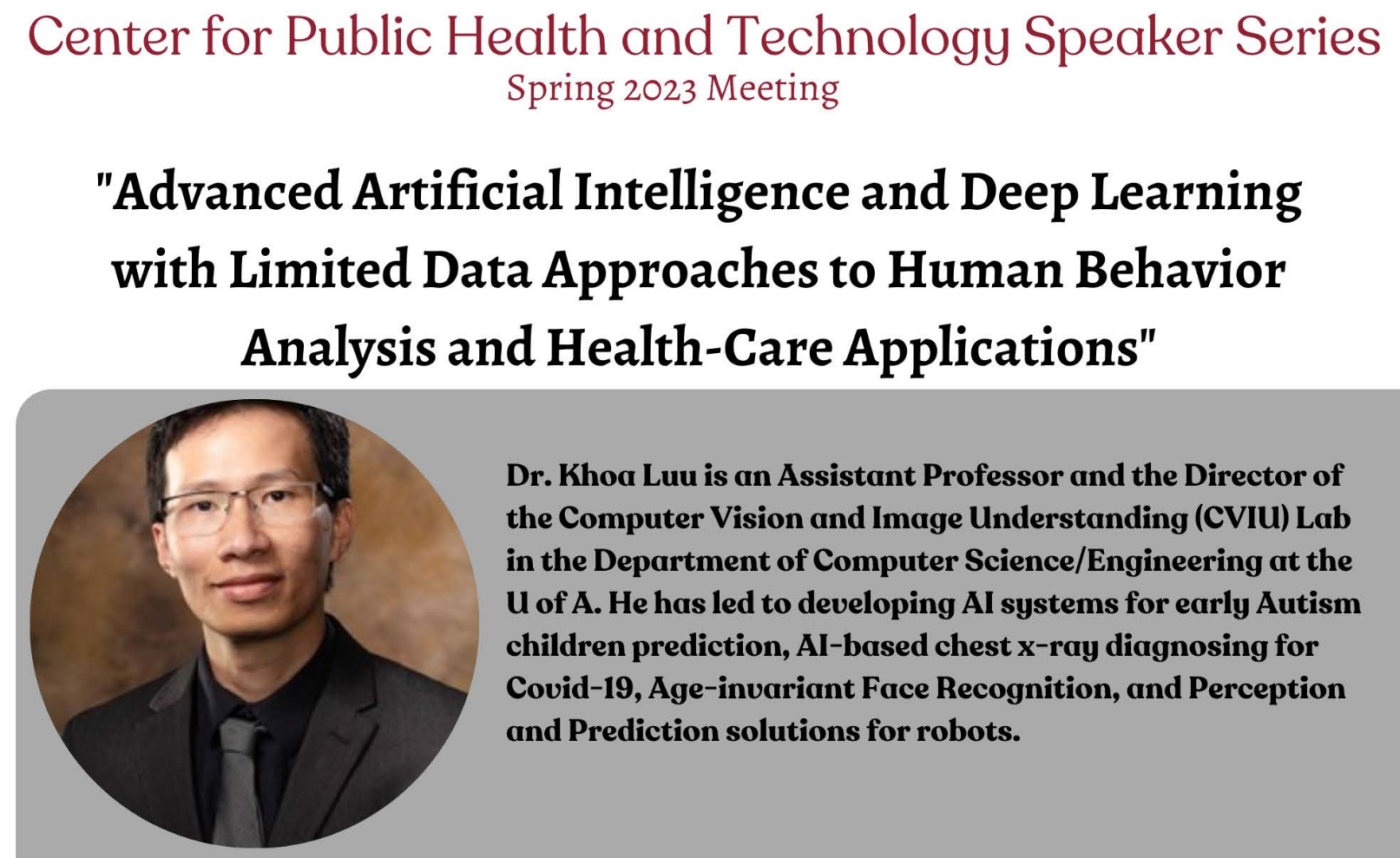
Advanced Artificial Intelligence and Deep Learning with Limited Data Approaches to Human Behavior Analysis and Health-Care Applications
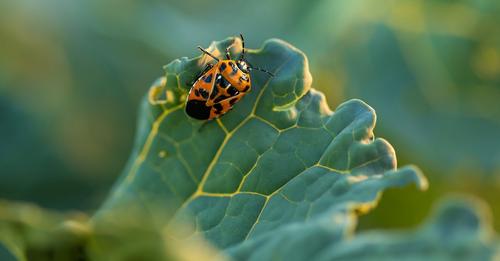
Short Takes: Caught on Camera: Insects Edition
University of Arkansas researchers have developed a prototype of an insect trap that can help farmers monitor and identify potential pests more efficiently in order to protect valuable crops. The trap, developed by researchers Ashley Dowling and Khoa Luu, captures footage of insects, uses artificial intelligence to identify them and sends real-time data back to the farmers. It also eliminates the need for manual monitoring, allowing farmers to make decisions on the fly and take the appropriate measures to counteract potential damage.
Link
Link
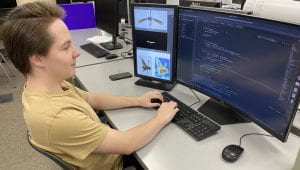
Training AI to Detect Crop Pests
Pierce Helton is an Honors student studying Computer Science. This summer, he worked as a research assistant in the department’s Computer Vision and Image Understanding Lab. Here, he had an opportunity to broaden his understanding of machine learning and contribute to department research. Pierce plans to work in the industry and potentially pursue a M.S. in Computer Science after earning his B.S.
Link
Link
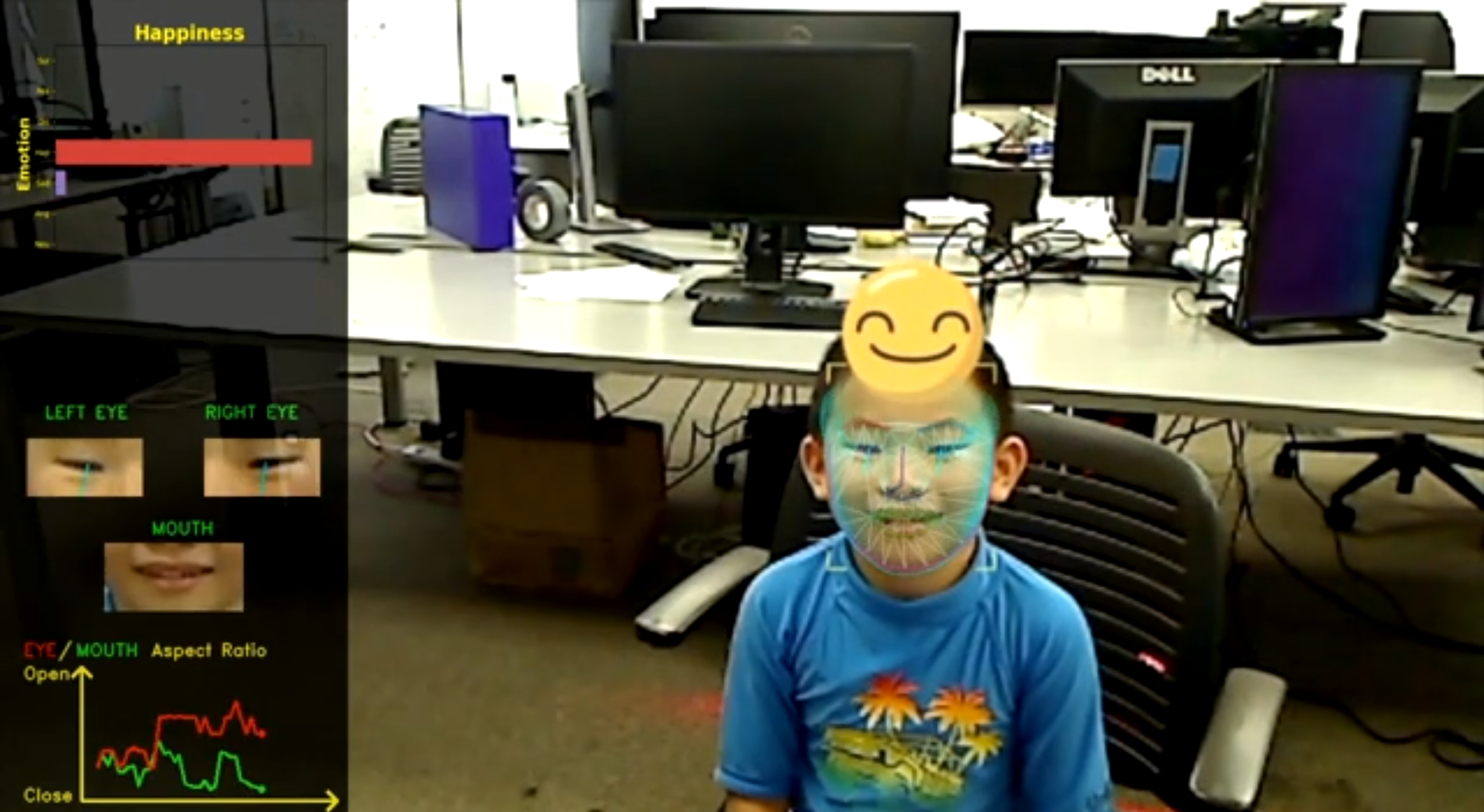
Researchers using artificial intelligence to assist with early detection of autism spectrum disorder
Faculty in food science and computer science/computer engineering are collaborating to develop machine learning that can assist in the detection of autism spectrum disorder.
Link
Link

MonArk NSF Quantum Foundry Established With $20 Million Grant
With a $20 million grant from the National Science Foundation, the U of A and Montana State University will establish the MonArk NSF Quantum Foundry to accelerate the development of quantum materials and devices.
Link
Link
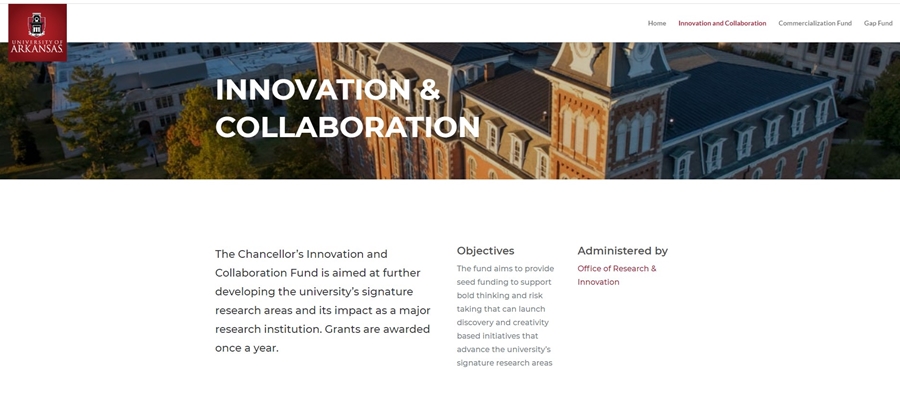
Chancellor's Innovation and Collaboration Fund Awards 10 Faculty Research Projects
In a continuous effort to promote research activity, ten faculty research projects have been awarded grants from the Chancellor's Innovation and Collaboration Fund.
Link
Link
This new AI tool ages faces in videos with creepy accuracy
A new machine learning paper shows how AI can take footage of someone and duplicate the video with the subject looking an age the researchers specify. The team behind the paper, from the University of Arkansas, Clemson University, Carnegie Mellon University, and Concordia University in Canada, claim that this is one of the first methods to use AI to tackle aging in videos.
Link
Link
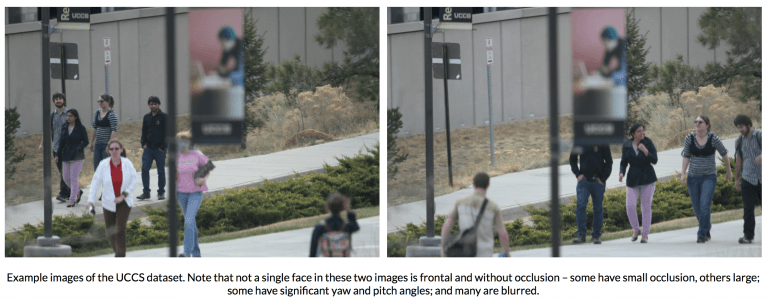
ECCV 2018 - 2nd Unconstrained Face Detection and Open Set Recognition Challenge
