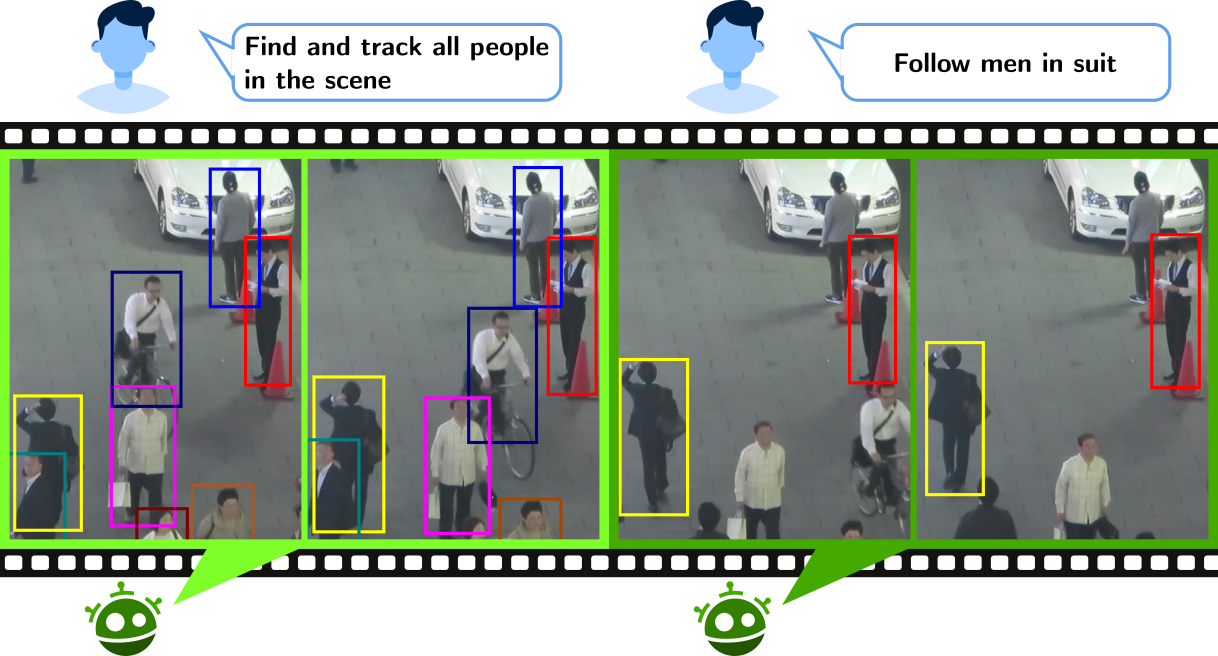
Type-to-Track: Retrieve Any Object via Prompt-based Tracking
Project Page | Paper | Poster | Video
One of the recent trends in vision problems is to use natural language captions to describe the objects of interest. This approach can overcome some limitations of traditional methods that rely on bounding boxes or category annotations. This paper introduces a novel paradigm for Multiple Object Tracking called Type-to-Track, which allows users to track objects in videos by typing natural language descriptions. We present a new dataset for that Grounded Multiple Object Tracking task, called GroOT, that contains videos with various types of objects and their corresponding textual captions describing their appearance and action in detail. Additionally, we introduce two new evaluation protocols and formulate evaluation metrics specifically for this task. We develop a new efficient method that models a transformer-based eMbed-ENcoDE-extRact framework (MENDER) using the third-order tensor decomposition. The experiments in five scenarios show that our MENDER approach outperforms another two-stage design in terms of accuracy and efficiency, up to 14.7% accuracy and 4× speed faster.
Nguyen, Pha, Kha Gia Quach, Kris Kitani, and Khoa Luu. "Type-to-Track: Retrieve Any Object via Prompt-based Tracking." Advances in Neural Information Processing Systems, 36. 2023.
@article{nguyen2023type, title = {Type-to-Track: Retrieve Any Object via Prompt-based Tracking}, author = {Nguyen, Pha and Quach, Kha Gia and Kitani, Kris and Luu, Khoa}, journal = {Advances in Neural Information Processing Systems}, volume = {36}, year = 2023}
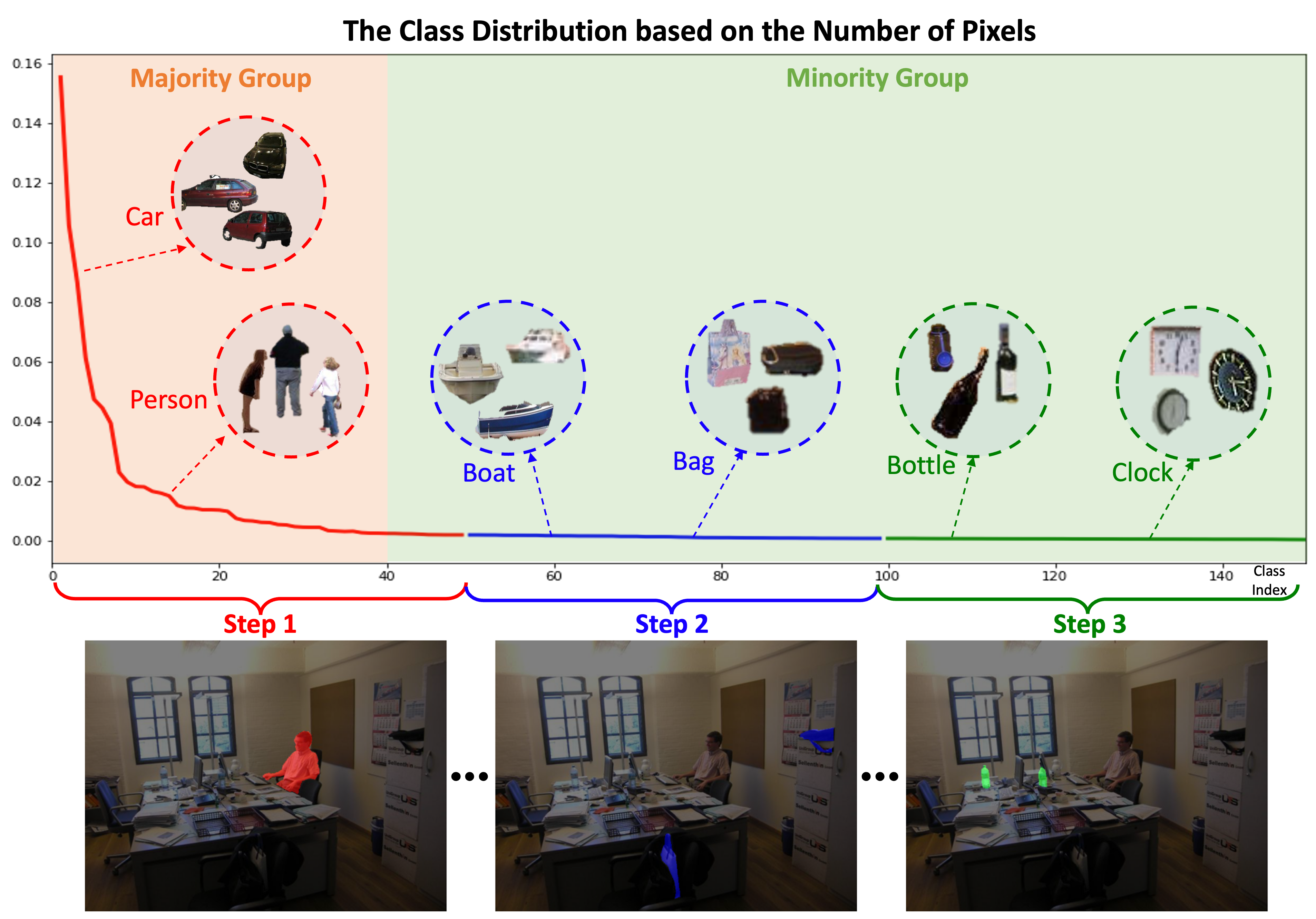
Fairness Continual Learning Approach to Semantic Scene Understanding in Open-World Environments
Paper
Continual semantic segmentation aims to learn new classes while maintaining the information from the previous classes. Although prior studies have shown impressive progress in recent years, the fairness concern in the continual semantic segmentation needs to be better addressed. Meanwhile, fairness is one of the most vital factors in deploying the deep learning model, especially in human-related or safety applications. In this paper, we present a novel Fairness Continual Learning approach to the semantic segmentation problem. In particular, under the fairness objective, a new fairness continual learning framework is proposed based on class distributions. Then, a novel Prototypical Contrastive Clustering loss is proposed to address the significant challenges in continual learning, i.e., catastrophic forgetting and background shift. Our proposed loss has also been proven as a novel, generalized learning paradigm of knowledge distillation commonly used in continual learning. Moreover, the proposed Conditional Structural Consistency loss further regularized the structural constraint of the predicted segmentation. Our proposed approach has achieved State-of-the-Art performance on three standard scene understanding benchmarks, i.e., ADE20K, Cityscapes, and Pascal VOC, and promoted the fairness of the segmentation model.
Thanh-Dat Truong, Hoang-Quan Nguyen, Bhiksha Raj, and Khoa Luu (2023). Fairness Continual Learning Approach to Semantic Scene Understanding in Open-World Environments. Thirty-seventh Conference on Neural Information Processing Systems (NeurIPS), 2023.
@inproceedings{ truong2023fairness, title={Fairness Continual Learning Approach to Semantic Scene Understanding in Open-World Environments}, author={Thanh-Dat Truong and Hoang-Quan Nguyen and Bhiksha Raj and Khoa Luu}, booktitle={Thirty-seventh Conference on Neural Information Processing Systems}, year={2023}, url={https://openreview.net/forum?id=KQ25VgEvOJ} }
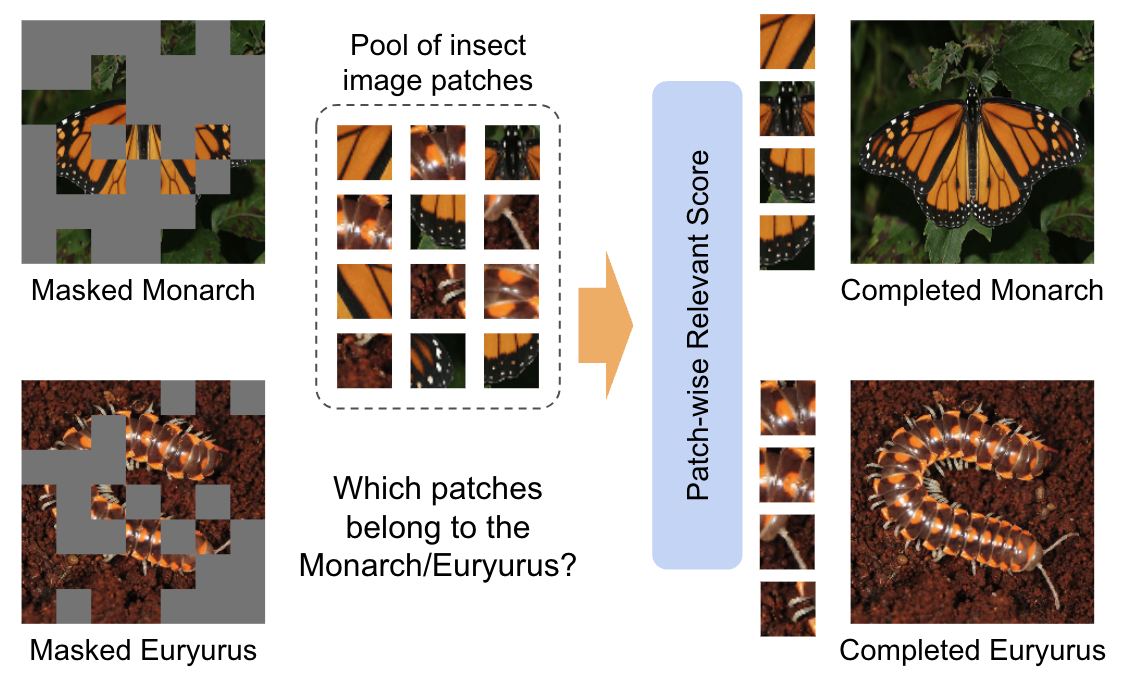
Insect-Foundation: A Foundation Model and Large-scale 1M Dataset for Visual Insect Understanding
Paper
In precision agriculture, the detection and recognition of insects play an essential role in the ability of crops to grow healthy and produce a high-quality yield. The current machine vision model requires a large volume of data to achieve high performance. However, there are approximately 5.5 million different insect species in the world. None of the existing insect datasets can cover even a fraction of them due to varying geographic locations and acquisition costs. In this paper, we introduce a novel ``Insect-1M'' dataset, a game-changing resource poised to revolutionize insect-related foundation model training. Covering a vast spectrum of insect species, our dataset, including 1 million images with dense identification labels of taxonomy hierarchy and insect descriptions, offers a panoramic view of entomology, enabling foundation models to comprehend visual and semantic information about insects like never before. Then, to efficiently establish an Insect Foundation Model, we develop a micro-feature self-supervised learning method with a Patch-wise Relevant Attention mechanism capable of discerning the subtle differences among insect images. In addition, we introduce Description Consistency loss to improve micro-feature modeling via insect descriptions. Through our experiments, we illustrate the effectiveness of our proposed approach in insect modeling and achieve State-of-the-Art performance on standard benchmarks of insect-related tasks. Our Insect Foundation Model and Dataset promise to empower the next generation of insect-related vision models, bringing them closer to the ultimate goal of precision agriculture.
Hoang-Quan Nguyen, Thanh-Dat Truong, Xuan Bac Nguyen, Ashley Dowling, Xin Li, and Khoa Luu (2023). Insect-Foundation: A Foundation Model and Large-scale 1M Dataset for Visual Insect Understanding. arXiv, 2023.
@article{nguyen2023insect, title={Insect-Foundation: A Foundation Model and Large-scale 1M Dataset for Visual Insect Understanding}, author={Nguyen, Hoang-Quan and Truong, Thanh-Dat and Nguyen, Xuan Bac and Dowling, Ashley and Li, Xin and Luu, Khoa}, journal={arXiv preprint arXiv:2311.15206}, year={2023} }
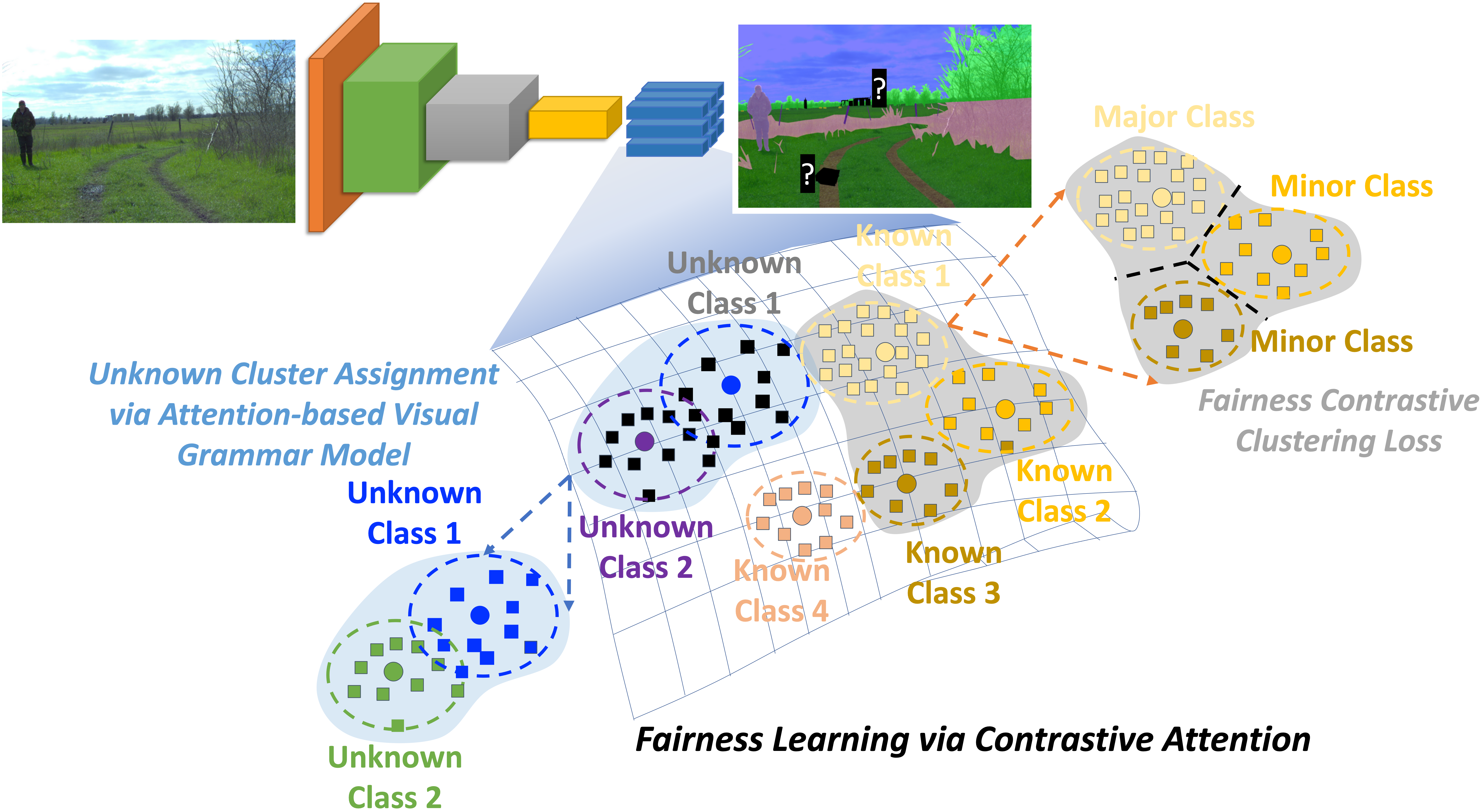
FALCON: Fairness Learning via Contrastive Attention Approach to Continual Semantic Scene Understanding in Open World
Paper
Continual Learning in semantic scene segmentation aims to continually learn new unseen classes in dynamic environments while maintaining previously learned knowledge. Prior studies focused on modeling the catastrophic forgetting and background shift challenges in continual learning. However, fairness, another major challenge that causes unfair predictions leading to low performance among major and minor classes, still needs to be well addressed. In addition, prior methods have yet to model the unknown classes well, thus resulting in producing non-discriminative features among unknown classes. This paper presents a novel Fairness Learning via Contrastive Attention Approach to continual learning in semantic scene understanding. In particular, we first introduce a new Fairness Contrastive Clustering loss to address the problems of catastrophic forgetting and fairness. Then, we propose an attention-based visual grammar approach to effectively model the background shift problem and unknown classes, producing better feature representations for different unknown classes. Through our experiments, our proposed approach achieves State-of-the-Art (SOTA) performance on different continual learning settings of three standard benchmarks, i.e., ADE20K, Cityscapes, and Pascal VOC. It promotes the fairness of the continual semantic segmentation model.
Thanh-Dat Truong, Utsav Prabhu, Bhiksha Raj, Jackson Cothren, and Khoa Luu (2023). FALCON: Fairness Learning via Contrastive Attention Approach to Continual Semantic Scene Understanding in Open World. arXiv, 2023.
@article{truong2023falcon, title={FALCON: Fairness Learning via Contrastive Attention Approach to Continual Semantic Scene Understanding in Open World}, author={Truong, Thanh-Dat and Prabhu, Utsav and Raj, Bhiksha and Cothren, Jackson and Luu, Khoa}, journal={arXiv preprint arXiv:2311.15965}, year={2023} }
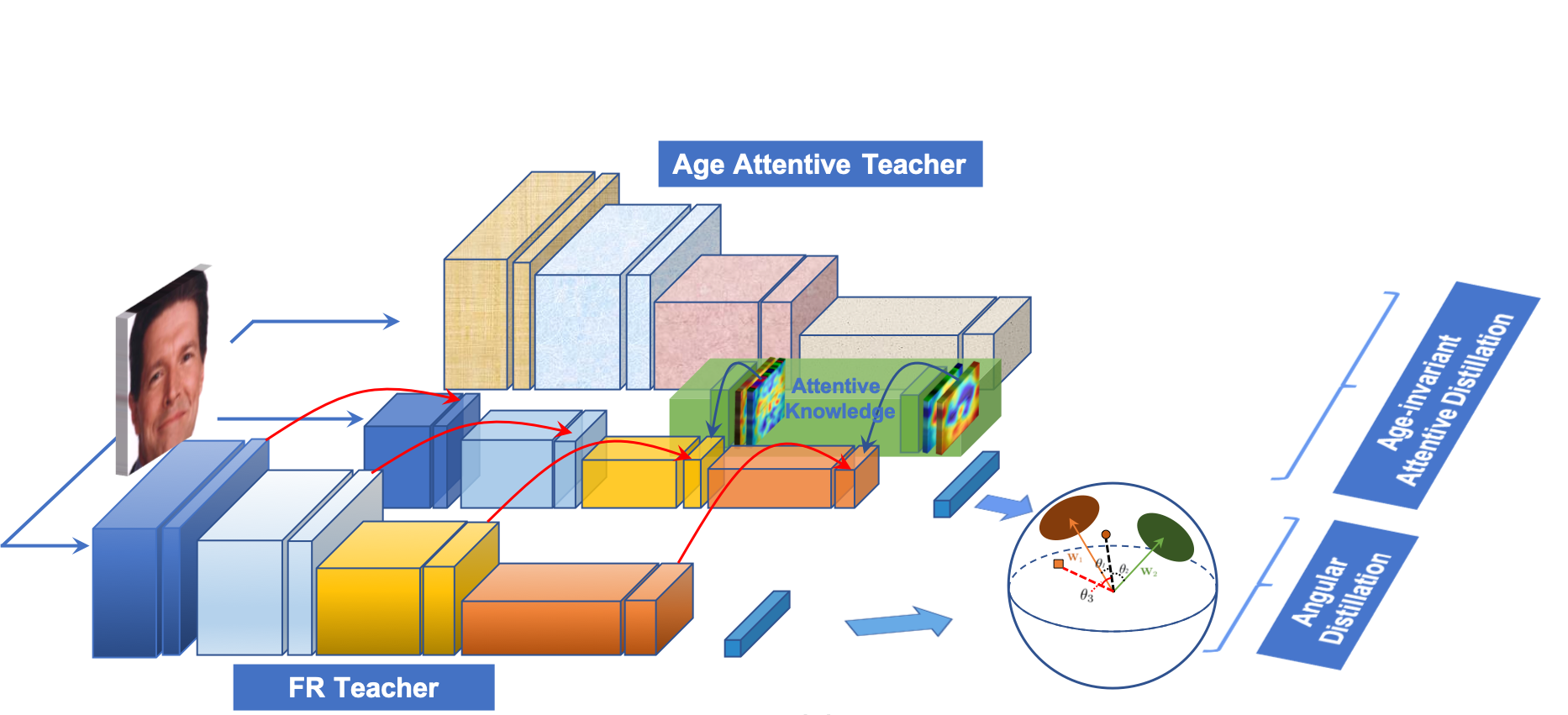
LIAAD: Lightweight Attentive Angular Distillation for Large-scale Age-Invariant Face Recognition
Paper
Disentangled representations have been commonly adopted to Age-invariant Face Recognition (AiFR) tasks. However, these methods have reached some limitations with (1) the requirement of large-scale face recognition (FR) training data with age labels, which is limited in practice; (2) heavy deep network architectures for high performance; and (3) their evaluations are usually taken place on age-related face databases while neglecting the standard large-scale FR databases to guarantee robustness. This work presents a novel Lightweight Attentive Angular Distillation (LIAAD) approach to Large-scale Lightweight AiFR that overcomes these limitations. Given two high-performance heavy networks as teachers with different specialized knowledge, LIAAD introduces a learning paradigm to efficiently distill the age-invariant attentive and angular knowledge from those teachers to a lightweight student network making it more powerful with higher FR accuracy and robust against age factor. Consequently, LIAAD approach is able to take the advantages of both FR datasets with and without age labels to train an AiFR model. Far apart from prior distillation methods mainly focusing on accuracy and compression ratios in closed-set problems, our LIAAD aims to solve the open-set problem, i.e. large-scale face recognition. Evaluations on LFW, IJB-B and IJB-C Janus, AgeDB and MegaFace-FGNet with one million distractors have demonstrated the efficiency of the proposed approach on light-weight structure. This work also presents a new longitudinal face aging (LogiFace) database for further studies in age-related facial problems in future.
Thanh-Dat Truong, Chi Nhan Duong, Kha Gia Quach, Ngan Le, Tien D Bui, and Khoa Luu (2023). LIAAD: Lightweight Attentive Angular Distillation for Large-scale Age-Invariant Face Recognition. Neurocomputing, 2023.
@article{truong2023liaad, title={LIAAD: Lightweight Attentive Angular Distillation for Large-scale Age-Invariant Face Recognition}, author={Thanh-Dat Truong and Chi Nhan Duong and Kha Gia Quach and Ngan Le and Tien D Bui and and Khoa Luu}, journal={Neurocomputing}, year={2023} }
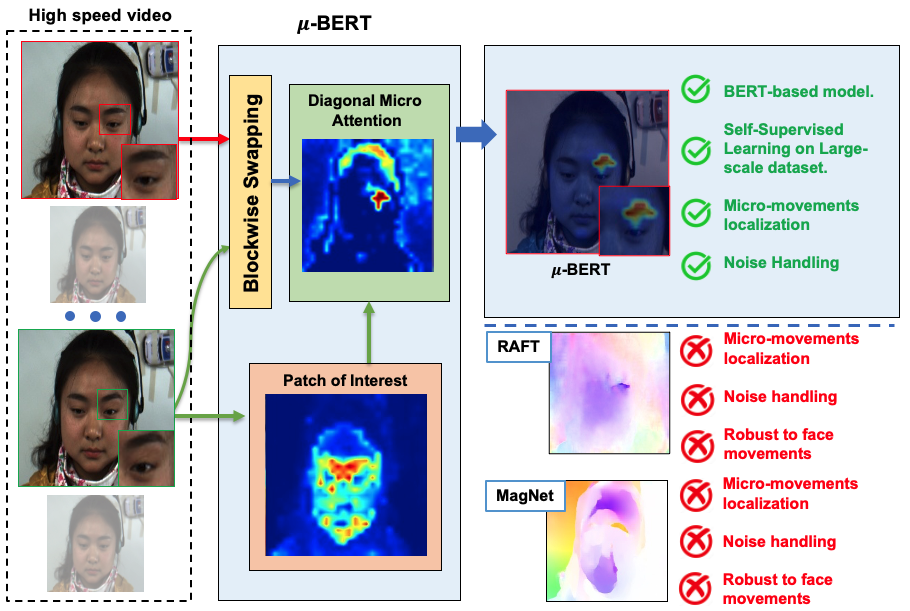
Micron-BERT: BERT-based Facial Micro-Expression Recognition
Micro-expression recognition is one of the most challenging topics in affective computing. It aims to recognize tiny facial movements difficult for humans to perceive in a brief period, i.e., 0.25 to 0.5 seconds. Recent advances in pre-training deep Bidirectional Transformers (BERT) have significantly improved self-supervised learning tasks in computer vision. However, the standard BERT in vision problems is designed to learn only from full images or videos, and the architecture cannot accurately detect details of facial micro-expressions. This paper presents Micron-BERT, a novel approach to facial micro-expression recognition. The proposed method can automatically capture these movements in an unsupervised manner based on two key ideas. First, we employ Diagonal Micro-Attention (DMA) to detect tiny differences between two frames. Second, we introduce a new Patch of Interest (PoI) module to localize and highlight micro-expression interest regions and simultaneously reduce noisy backgrounds and distractions. By incorporating these components into an end-to-end deep network, the proposed -BERT significantly outperforms all previous work in various micro-expression tasks. -BERT can be trained on a large-scale unlabeled dataset, i.e., up to 8 million images, and achieves high accuracy on new unseen facial micro-expression datasets. Empirical experiments show -BERT consistently outperforms state-of-the-art performance on four micro-expression benchmarks, including SAMM, CASME II, SMIC, and CASME3, by significant margins. Code will be available at https://github.com/uark-cviu/Micron-BERT.
Nguyen, Xuan-Bac and Duong, Chi Nhan and Xin, Li and Susan, Gauch and Han-Seok, Seo and Luu, Khoa (2023). Micron-BERT: BERT-based Facial Micro-Expression Recognition.
@INPROCEEDINGS{nguyen2023micronbert, author={Nguyen, Xuan-Bac and Duong, Chi Nhan and Xin, Li and Susan, Gauch and Han-Seok, Seo and Luu, Khoa}, booktitle={2023 IEEE/CVF Conference on Computer Vision and Pattern Recognition (CVPR)}, title={Micron-BERT: BERT-based Facial Micro-Expression Recognition}, year={2023} }
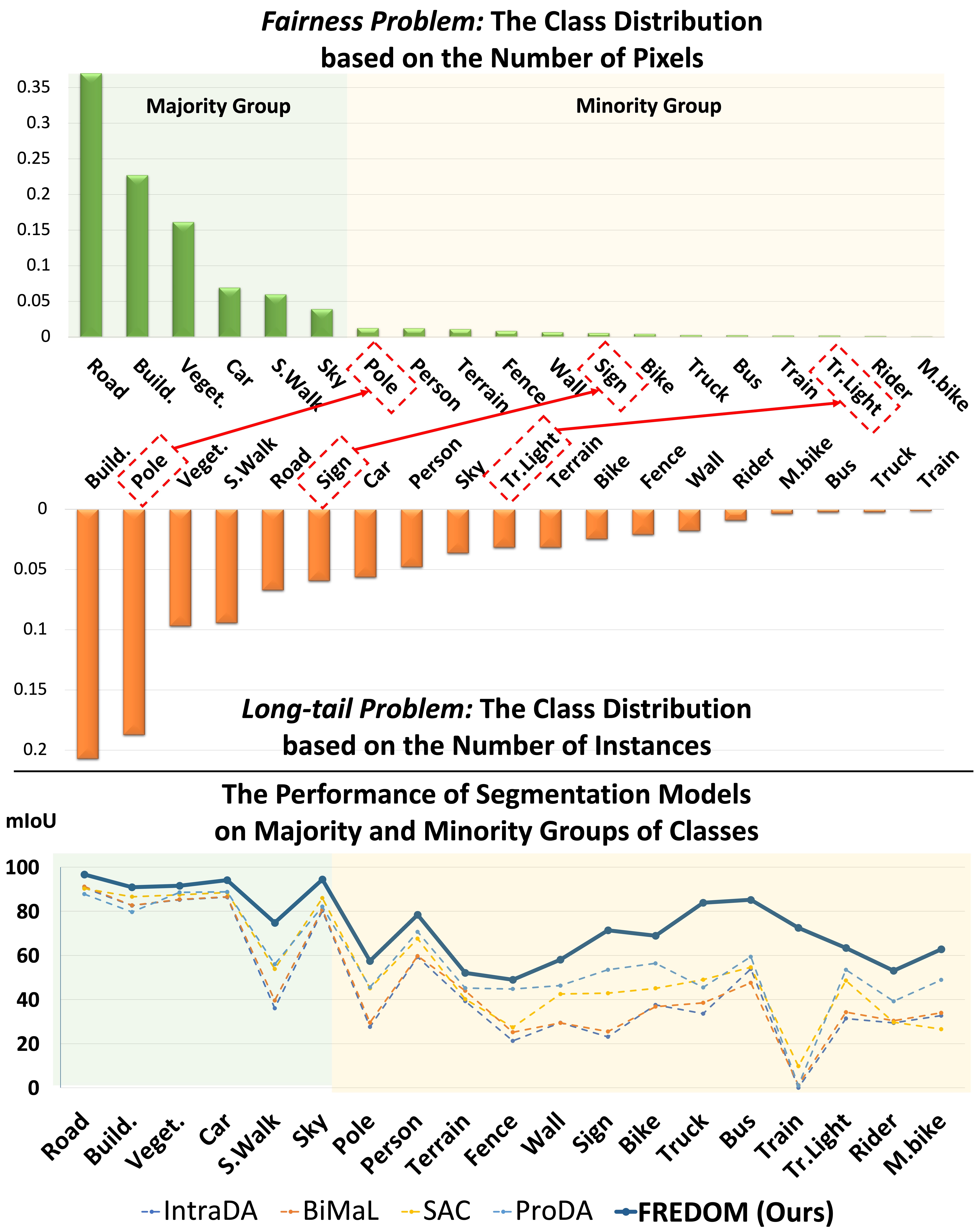
FREDOM: Fairness Domain Adaptation Approach to Semantic Scene Understanding
Paper | Video
Although Domain Adaptation in Semantic Scene Segmentation has shown impressive improvement in recent years, the fairness concerns in the domain adaptation have yet to be well defined and addressed. In addition, fairness is one of the most critical aspects when deploying the segmentation models into human-related real-world applications, e.g., autonomous driving, as any unfair predictions could influence human safety. In this paper, we propose a novel Fairness Domain Adaptation (FREDOM) approach to semantic scene segmentation. In particular, from the proposed formulated fairness objective, a new adaptation framework will be introduced based on the fair treatment of class distributions. Moreover, to generally model the context of structural dependency, a new conditional structural constraint is introduced to impose the consistency of predicted segmentation. Thanks to the proposed Conditional Structure Network, the self-attention mechanism has sufficiently modeled the structural information of segmentation. Through the ablation studies, the proposed method has shown the performance improvement of the segmentation models and promoted fairness in the model predictions. The experimental results on the two standard benchmarks, i.e., SYNTHIA to Cityscapes and GTA5 to Cityscapes, have shown that our method achieved State-of-the-Art (SOTA) performance.
Thanh-Dat Truong, Ngan Le, Bhiksha Raj, Jackson Cothren, and Khoa Luu (2023). FREDOM: Fairness Domain Adaptation Approach to Semantic Scene Understanding. In Proceedings of the IEEE/CVF Conference on Computer Vision and Pattern Recognition (CVPR), 2023.
@article{truong2023freedom, title={FREDOM: Fairness Domain Adaptation Approach to Semantic Scene Understanding}, author={Thanh-Dat Truong and Ngan Le and Bhiksha Raj and Jackson Cothren and Khoa Luu}, journal={IEEE/CVF Conference on Computer Vision and Pattern Recognition (CVPR)}, year={2023} }
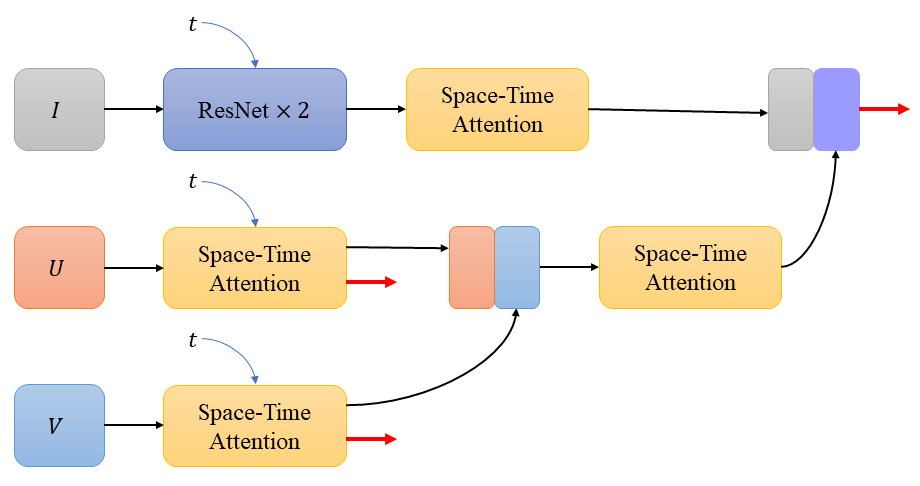
Neural Cell Video Synthesis via Optical-Flow Diffusion
Paper
The biomedical imaging world is notorious for working with small amounts of data, frustrating state-of-the-art efforts in the computer vision and deep learning worlds. With large datasets, it is easier to make progress we have seen from the natural image distribution. It is the same with microscopy videos of neuron cells moving in a culture. This problem presents several challenges as it can be difficult to grow and maintain the culture for days, and it is expensive to acquire the materials and equipment. In this work, we explore how to alleviate this data scarcity problem by synthesizing the videos. We, therefore, take the recent work of the video diffusion model to synthesize videos of cells from our training dataset. We then analyze the model's strengths and consistent shortcomings to guide us on improving video generation to be as high-quality as possible. To improve on such a task, we propose modifying the denoising function and adding motion information (dense optical flow) so that the model has more context regarding how video frames transition over time and how each pixel changes over time.
Serna-Aguilera, Manuel, Khoa Luu, Nathaniel Harris, and Min Zou. "Neural Cell Video Synthesis via Optical-Flow Diffusion." arXiv preprint arXiv:2212.03250 (2022).
@article{serna2022neural, title={Neural Cell Video Synthesis via Optical-Flow Diffusion}, author={Serna-Aguilera, Manuel and Luu, Khoa and Harris, Nathaniel and Zou, Min}, journal={arXiv preprint arXiv:2212.03250}, year={2022} }
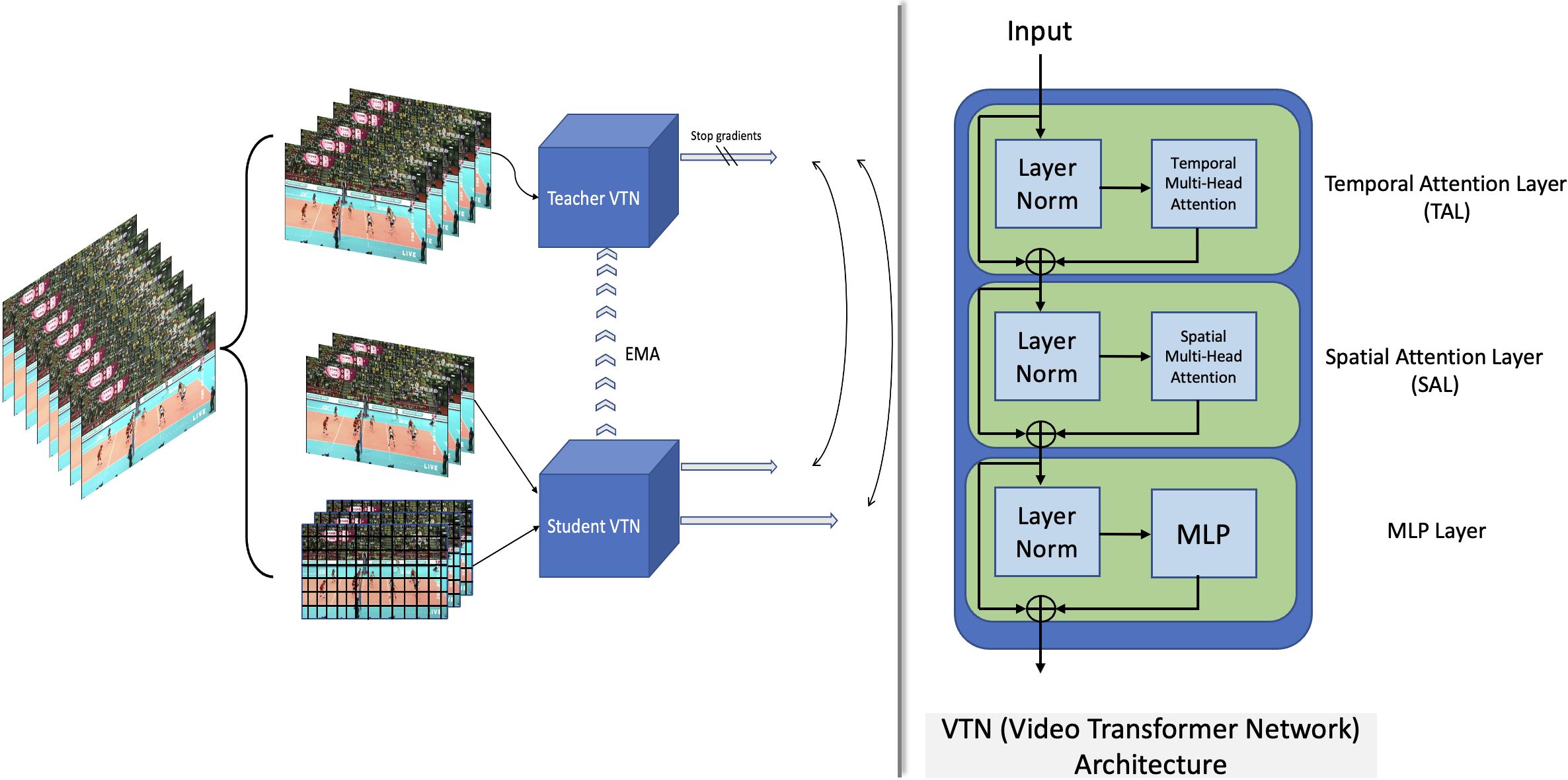
SPARTAN: Self-supervised Spatiotemporal Transformers Approach to Group Activity Recognition
Paper
In this paper, we propose a new, simple, and effective Self-supervised Spatio-temporal Transformers (SPARTAN) approach to Group Activity Recognition (GAR) using unlabeled video data. Given a video, we create local and global Spatio-temporal views with varying spatial patch sizes and frame rates. The proposed self-supervised objective aims to match the features of these contrasting views representing the same video to be consistent with the variations in spatiotemporal domains. To the best of our knowledge, the proposed mechanism is one of the first works to alleviate the weakly supervised setting of GAR using the encoders in video transformers. Furthermore, using the advantage of transformer models, our proposed approach supports long-term relationship modeling along spatio-temporal dimensions. The proposed SPARTAN approach performs well on two group activity recognition benchmarks, including NBA and Volleyball datasets, by surpassing the state-of-the-art results by a significant margin in terms of MCA and MPCA metrics.
Chappa, Naga VS, Pha Nguyen, Alexander H. Nelson, Han-Seok Seo, Xin Li, Page Daniel Dobbs, and Khoa Luu. "Group Activity Recognition using Self-supervised Approach of Spatiotemporal Transformers." arXiv preprint arXiv:2303.12149 (2023).
@article{chappa2023group, title={Group Activity Recognition using Self-supervised Approach of Spatiotemporal Transformers}, author={Chappa, Naga VS and Nguyen, Pha and Nelson, Alexander H and Seo, Han-Seok and Li, Xin and Dobbs, Page Daniel and Luu, Khoa}, journal={arXiv preprint arXiv:2303.12149}, year={2023} }
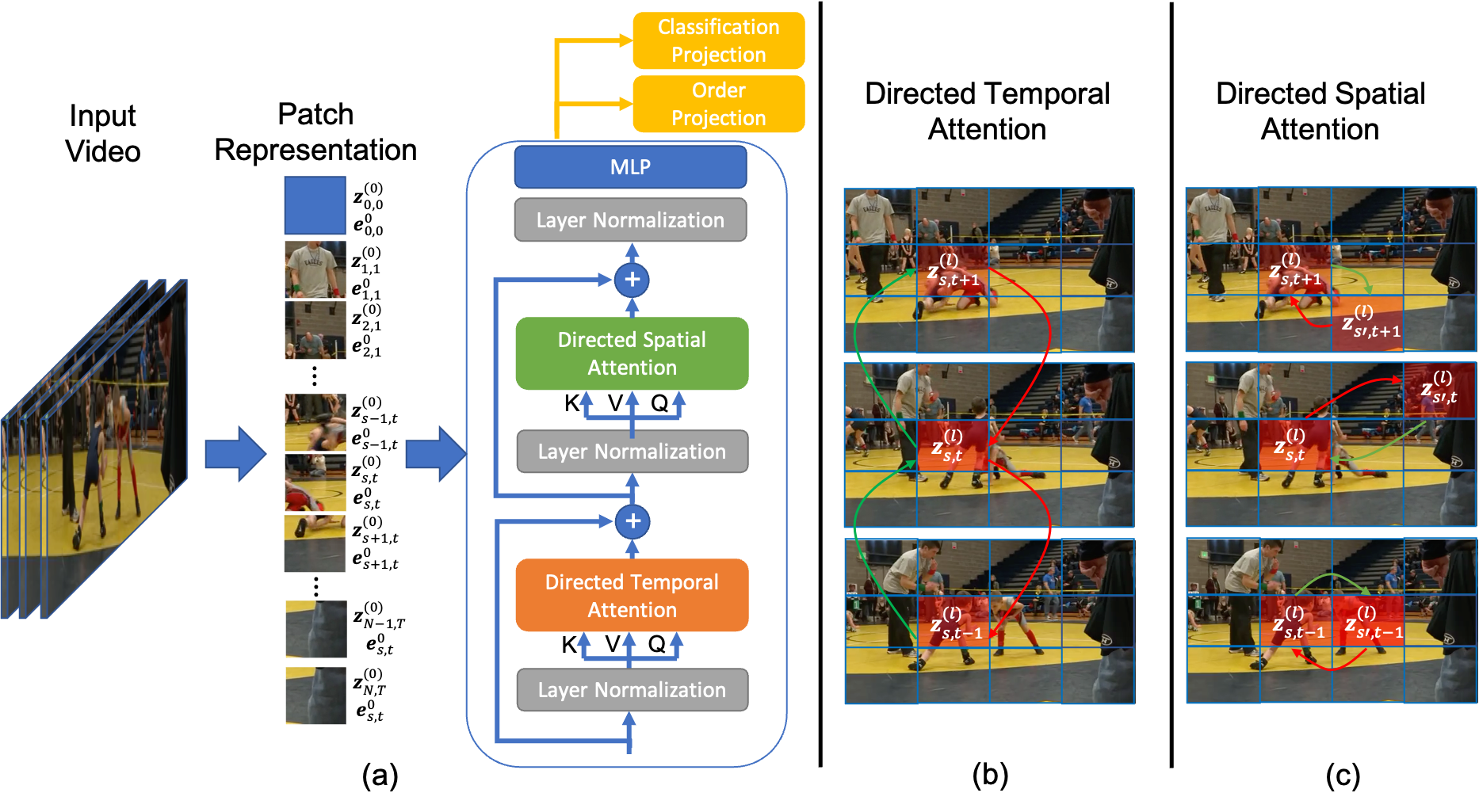
DirecFormer: A Directed Attention in Transformer Approach to Robust Action Recognition
Human action recognition has recently become one of the popular research topics in the computer vision community. Various 3D-CNN based methods have been presented to tackle both the spatial and temporal dimensions in the task of video action recognition with competitive results. However, these methods have suffered some fundamental limitations such as lack of robustness and generalization, e.g., how does the temporal ordering of video frames affect the recognition results? This work presents a novel end-to-end Transformer-based Directed Attention (DirecFormer) framework for robust action recognition. The method takes a simple but novel perspective of Transformer-based approach to understand the right order of sequence actions. Therefore, the contributions of this work are three-fold. Firstly, we introduce the problem of ordered temporal learning issues to the action recognition problem. Secondly, a new Directed Attention mechanism is introduced to understand and provide attentions to human actions in the right order. Thirdly, we introduce the conditional dependency in action sequence modeling that includes orders and classes. The proposed approach consistently achieves the state-of-the-art (SOTA) results compared with the recent action recognition methods, on three standard large-scale benchmarks, i.e. Jester, Kinetics-400 and Something-Something-V2.
Thanh-Dat Truong, Quoc-Huy Bui, Chi Nhan Duong, Han-Seok Seo, Son Lam Phung, Xin Li, and Khoa Luu (2022). DirecFormer: A Directed Attention in Transformer Approach to Robust Action Recognition. In Proceedings of the IEEE/CVF Conference on Computer Vision and Pattern Recognition (CVPR), 2022.
@article{truong2022direcformer, title={DirecFormer: A Directed Attention in Transformer Approach to Robust Action Recognition}, author={Thanh-Dat Truong and Quoc-Huy Bui and Chi Nhan Duong and Han-Seok Seo and Son Lam Phung and Xin Lia and Khoa Luu}, journal={IEEE/CVF Conference on Computer Vision and Pattern Recognition (CVPR)}, year={2022} }
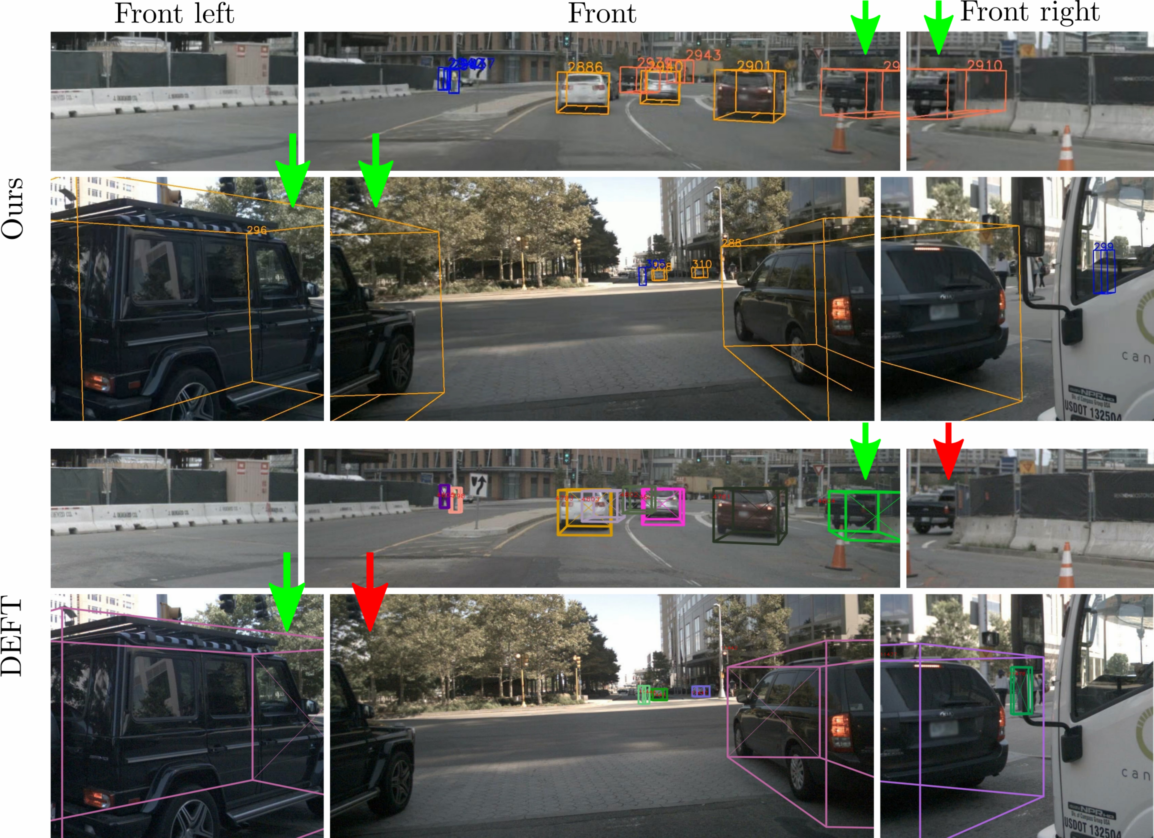
Multi-Camera Multiple 3D Object Tracking on the Move for Autonomous Vehicles
Paper | Poster | Video
The development of autonomous vehicles provides an opportunity to have a complete set of camera sensors capturing the environment around the car. Thus, it is important for object detection and tracking to address new challenges, such as achieving consistent results across views of cameras. To address these challenges, this work presents a new Global Association Graph Model with Link Prediction approach to predict existing tracklets location and link detections with tracklets via cross-attention motion modeling and appearance re-identification. This approach aims at solving issues caused by inconsistent 3D object detection. Moreover, our model exploits to improve the detection accuracy of a standard 3D object detector in the nuScenes detection challenge. The experimental results on the nuScenes dataset demonstrate the benefits of the proposed method to produce SOTA performance on the existing vision-based tracking dataset.
Nguyen, P., Quach, K. G., Duong, C. N., Phung, S. L., Le, N., & Luu, K. (2022). Multi-Camera Multi-Object Tracking on the Move via Single-Stage Global Association Approach. arXiv preprint arXiv:2211.09663.
@article{nguyen2022multi, title={Multi-Camera Multi-Object Tracking on the Move via Single-Stage Global Association Approach}, author={Nguyen, Pha and Quach, Kha Gia and Duong, Chi Nhan and Phung, Son Lam and Le, Ngan and Luu, Khoa}, journal={arXiv preprint arXiv:2211.09663}, year={2022} }
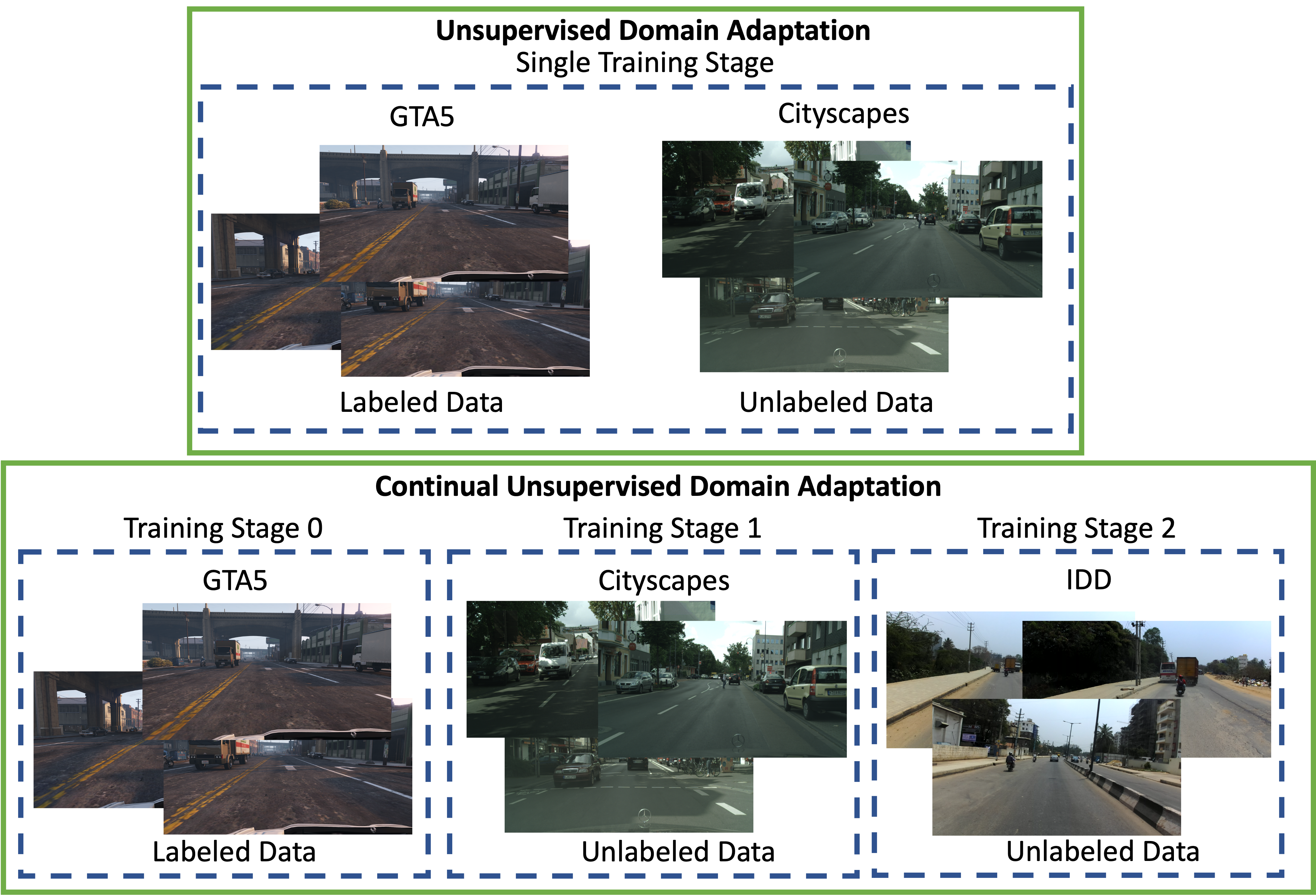
CONDA: Continual Unsupervised Domain Adaptation Learning in Visual Perception for Self-Driving Cars
Paper
Although unsupervised domain adaptation methods have achieved remarkable performance in semantic scene segmentation in visual perception for self-driving cars, these approaches remain impractical in real-world use cases. In practice, the segmentation models may encounter new data that have not been seen yet. Also, the previous data training of segmentation models may be inaccessible due to privacy problems. Therefore, to address these problems, in this work, we propose a Continual Unsupervised Domain Adaptation (CONDA) approach that allows the model to continuously learn and adapt with respect to the presence of the new data. Moreover, our proposed approach is designed without the requirement of accessing previous training data. To avoid the catastrophic forgetting problem and maintain the performance of the segmentation models, we present a novel Bijective Maximum Likelihood loss to impose the constraint of predicted segmentation distribution shifts. The experimental results on the benchmark of continual unsupervised domain adaptation have shown the advanced performance of the proposed CONDA method.
Thanh-Dat Truong, Pierce Helton, Ahmed Moustafa, Jackson David Cothren, and Khoa Luu (2022). CONDA: Continual Unsupervised Domain Adaptation Learning in Visual Perception for Self-Driving Cars. arXiv, 2022.
@article{truong2022conda, author={Thanh-Dat Truong and Pierce Helton and Ahmed Moustafa and Jackson David Cothren and Khoa Luu}, title = {CONDA: Continual Unsupervised Domain Adaptation Learning in Visual Perception for Self-Driving Cars}, journal = {arXiv}, year = {2022}}
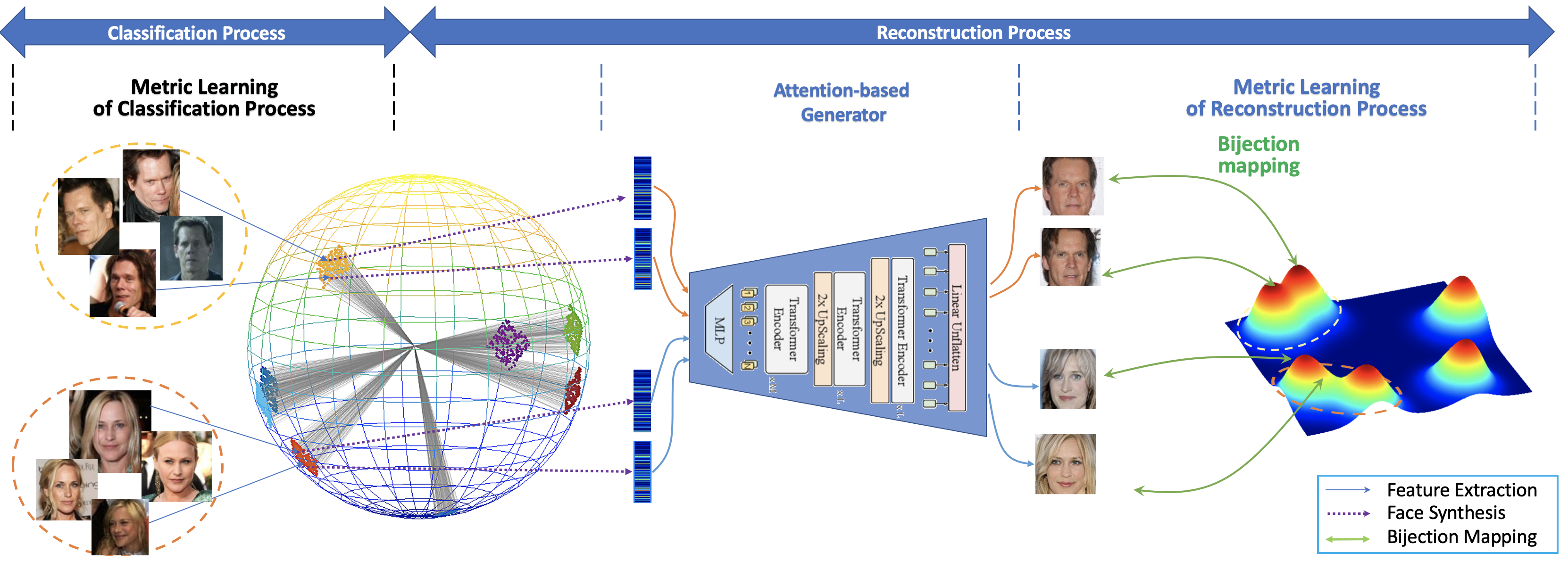
Vec2Face-v2: Unveil Human Faces from their Blackbox Features via Attention-based Network in Face Recognition
Paper
In this work, we investigate the problem of face reconstruction given a facial feature representation extracted from a blackbox face recognition engine. Indeed, it is very challenging problem in practice due to the limitations of abstracted information from the engine. We therefore introduce a new method named Attention-based Bijective Generative Adversarial Networks in a Distillation framework (DAB-GAN) to synthesize faces of a subject given his/her extracted face recognition features. Given any unconstrained unseen facial features of a subject, the DAB-GAN can reconstruct his/her faces in high definition. The DAB-GAN method includes a novel attention-based generative structure with the new defined Bijective Metrics Learning approach. The framework starts by introducing a bijective metric so that the distance measurement and metric learning process can be directly adopted in image domain for an image reconstruction task. The information from the blackbox face recognition engine will be optimally exploited using the global distillation process. Then an attention-based generator is presented for a highly robust generator to synthesize realistic faces with ID preservation. We have evaluated our method on the challenging face recognition databases, i.e. CelebA, LFW, AgeDB, CFP-FP, and consistently achieved the state-of-the-art results. The advancement of DAB-GAN is also proven on both image realism and ID preservation properties.
Truong, Thanh-Dat, Chi Nhan Duong, Ngan Le, Marios Savvides, and Khoa Luu. "Vec2Face-v2: Unveil Human Faces from their Blackbox Features via Attention-based Network in Face Recognition." arXiv preprint arXiv:2209.04920 (2022).
@article{truong2022vec2facev2, title={Vec2Face-v2: Unveil Human Faces from their Blackbox Features via Attention-based Network in Face Recognition}, author={Thanh-Dat Truong and Chi Nhan Duong and Ngan Le and Marios Savvides and Khoa Luu}, year={2022}, journal={arXiv}, }
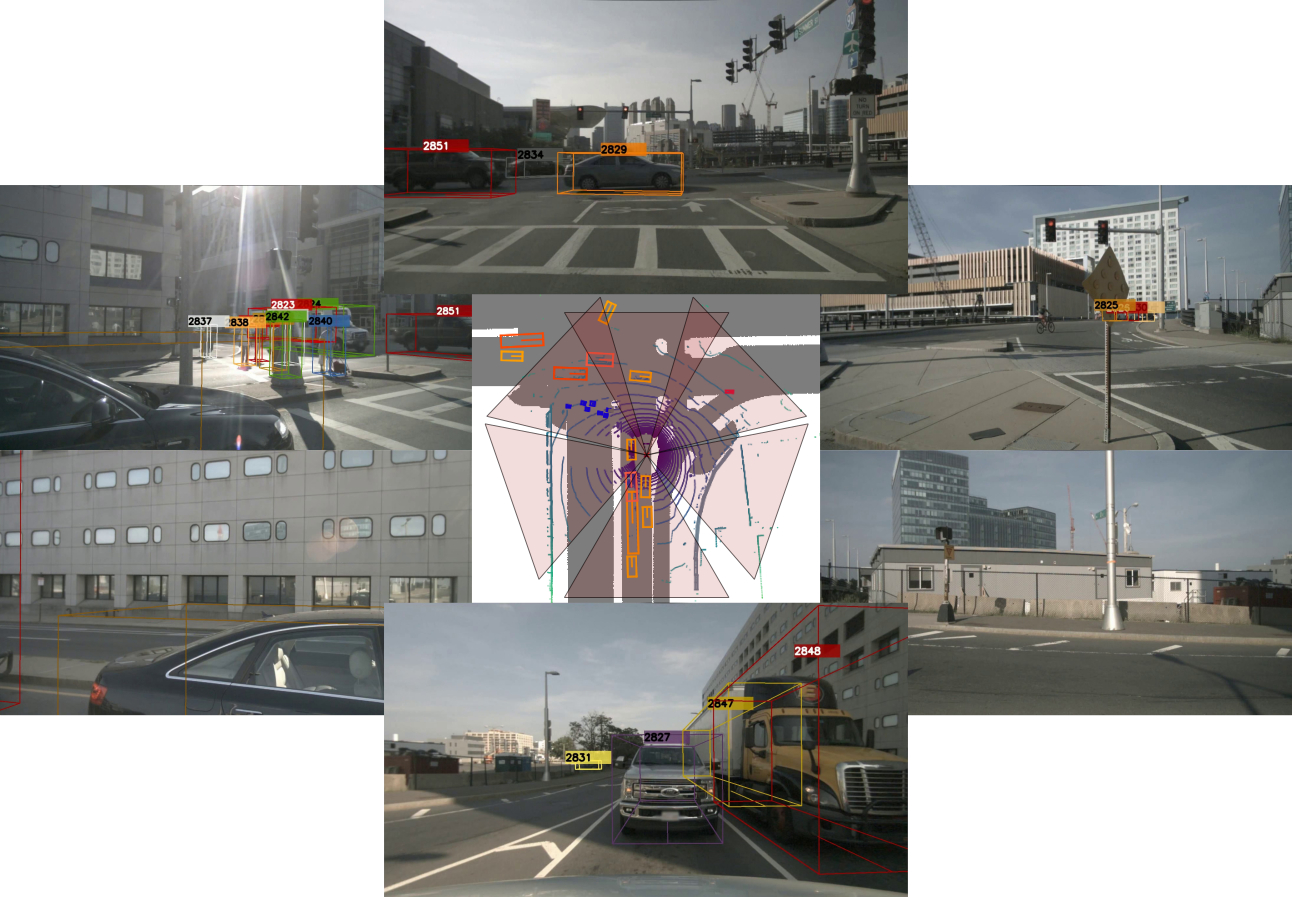
Multi-Camera Multi-Object Tracking on the Move via Single-Stage Global Association Approach
Paper
The development of autonomous vehicles generates a tremendous demand for a low-cost solution with a complete set of camera sensors capturing the environment around the car. It is essential for object detection and tracking to address these new challenges in multi-camera settings. In order to address these challenges, this work introduces novel Single-Stage Global Association Tracking approaches to associate one or more detection from multi-cameras with tracked objects. These approaches aim to solve fragment-tracking issues caused by inconsistent 3D object detection. Moreover, our models also improve the detection accuracy of the standard vision-based 3D object detectors in the nuScenes detection challenge. The experimental results on the nuScenes dataset demonstrate the benefits of the proposed method by outperforming prior vision-based tracking methods in multi-camera settings.
Nguyen, P., Quach, K. G., Duong, C. N., Phung, S. L., Le, N., & Luu, K. (2022). Multi-Camera Multi-Object Tracking on the Move via Single-Stage Global Association Approach. arXiv preprint arXiv:2211.09663.
@article{nguyen2022multi, title={Multi-Camera Multi-Object Tracking on the Move via Single-Stage Global Association Approach}, author={Nguyen, Pha and Quach, Kha Gia and Duong, Chi Nhan and Phung, Son Lam and Le, Ngan and Luu, Khoa}, journal={arXiv preprint arXiv:2211.09663}, year={2022} }
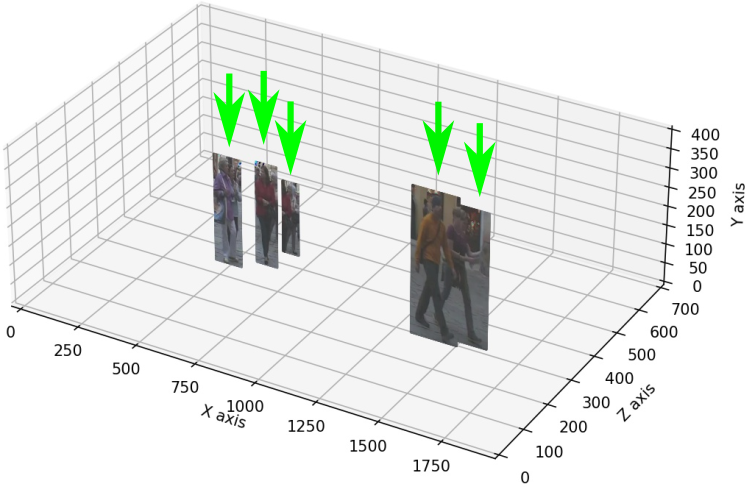
Depth Perspective-aware Multiple Object Tracking
Paper
This paper aims to tackle Multiple Object Tracking (MOT), an important problem in computer vision but remains challenging due to many practical issues, especially occlusions. Indeed, we propose a new real-time Depth Perspective-aware Multiple Object Tracking (DP-MOT) approach to tackle the occlusion problem in MOT. A simple yet efficient Subject-Ordered Depth Estimation (SODE) is first proposed to automatically order the depth positions of detected subjects in a 2D scene in an unsupervised manner. Using the output from SODE, a new Active pseudo-3D Kalman filter, a simple but effective extension of Kalman filter with dynamic control variables, is then proposed to dynamically update the movement of objects. In addition, a new high-order association approach is presented in the data association step to incorporate first-order and second-order relationships between the detected objects. The proposed approach consistently achieves state-of-the-art performance compared to recent MOT methods on standard MOT benchmarks.
Nguyen, P., Quach, K. G., Duong, C. N., Phung, S. L., Le, N., & Luu, K. (2022). Multi-Camera Multi-Object Tracking on the Move via Single-Stage Global Association Approach. arXiv preprint arXiv:2211.09663.
@article{nguyen2022multi, title={Multi-Camera Multi-Object Tracking on the Move via Single-Stage Global Association Approach}, author={Nguyen, Pha and Quach, Kha Gia and Duong, Chi Nhan and Phung, Son Lam and Le, Ngan and Luu, Khoa}, journal={arXiv preprint arXiv:2211.09663}, year={2022} }
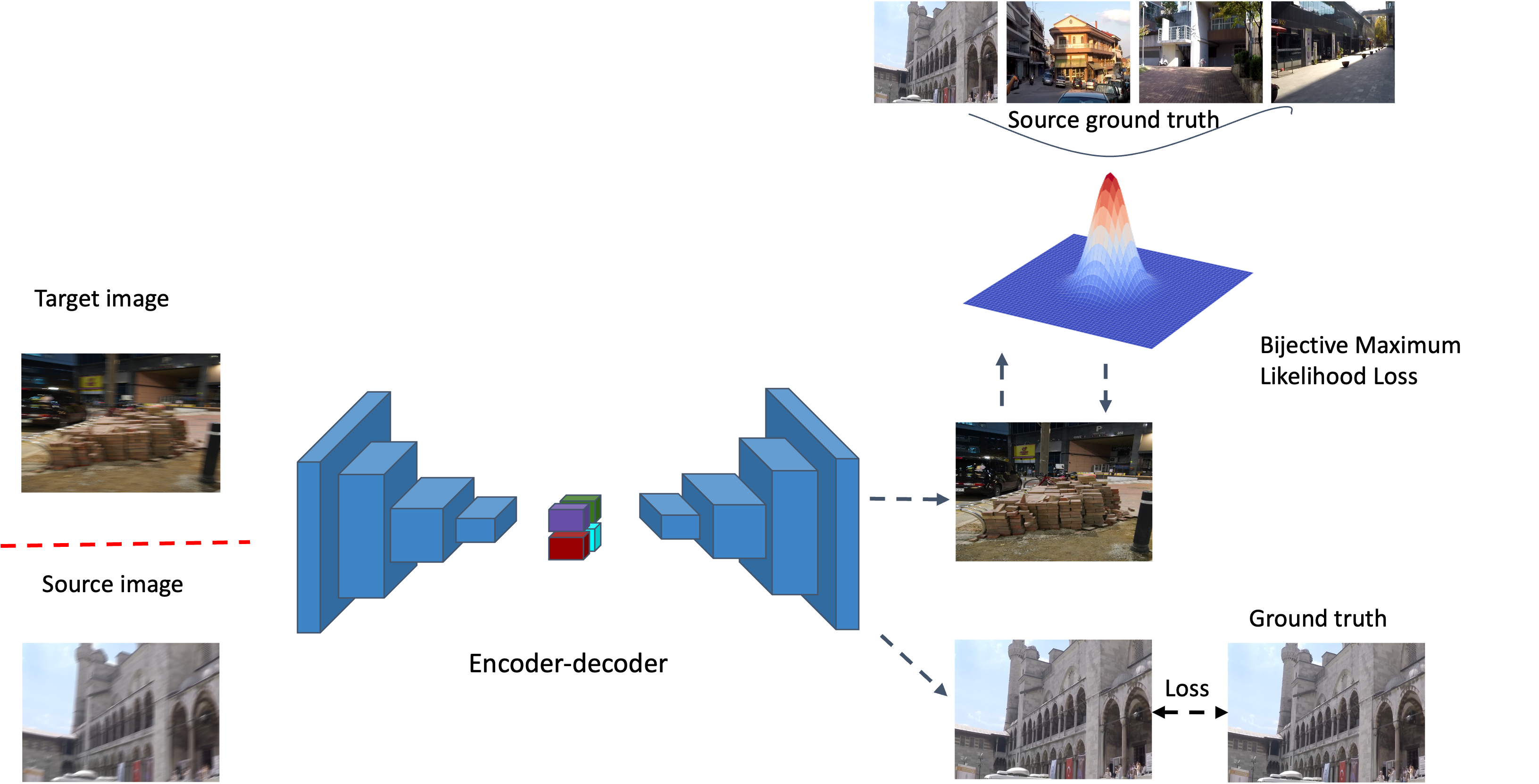
EQAdap: Equipollent Domain Adaptation Approach to Image Deblurring
Paper
In this paper, we present an end-to-end unsupervised domain adaptation approach to image deblurring. This work focuses on learning and generalizing the complex latent space of the source domain and transferring the extracted information to the unlabeled target domain. While fully supervised image deblurring methods have achieved high accuracy on large-scale vision datasets, they are unable to well generalize well on a new test environment or a new domain. Therefore, in this work, we introduce a novel Bijective Maximum Likelihood loss for the unsupervised domain adaptation approach to image deblurring. We evaluate our proposed method on GoPro, RealBlur_J, RealBlur_R, and HIDE datasets. Through intensive experiments, we demonstrate our state-of-the-art performance on the standard benchmarks.
Jalata, Ibsa, Naga Venkata Sai Raviteja Chappa, Thanh-Dat Truong, Pierce Helton, Chase Rainwater, and Khoa Luu. "EQAdap: Equipollent Domain Adaptation Approach to Image Deblurring." IEEE Access 10 (2022): 93203-93211.
@article{jalata2022eqadap, author={Jalata, Ibsa and Chappa, Naga Venkata Sai Raviteja and Truong, Thanh-Dat and Helton, Pierce and Rainwater, Chase and Luu, Khoa}, journal={IEEE Access}, title={EQAdap: Equipollent Domain Adaptation Approach to Image Deblurring}, year={2022}, volume={10}, pages={93203-93211}, doi={10.1109/ACCESS.2022.3203736}}
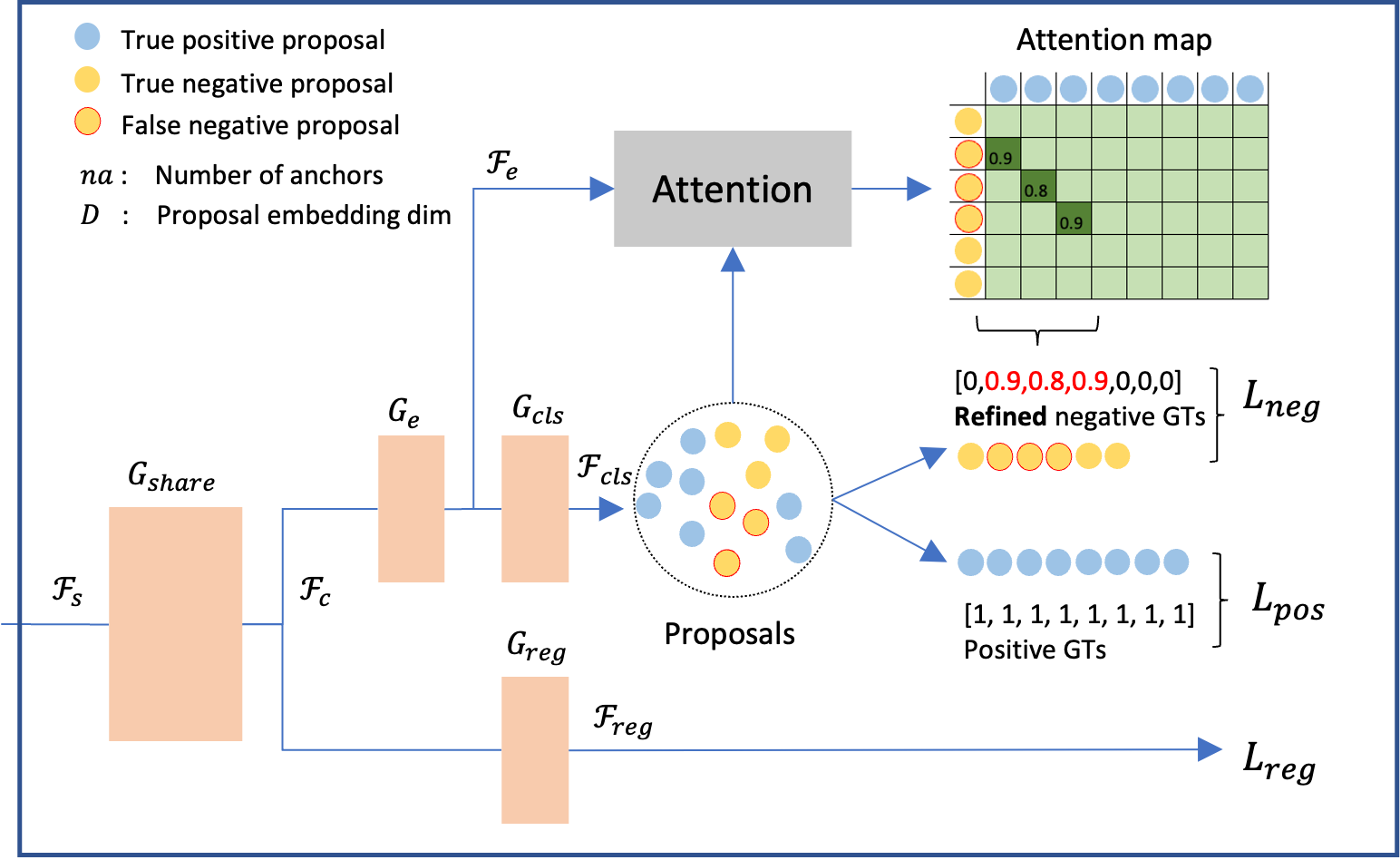
Two-dimensional quantum material identification via self-attention and soft-labeling in deep learning
Paper
In quantum machine field, detecting two-dimensional (2D) materials in Silicon chips is one of the most critical problems. Instance segmentation can be considered as a potential approach to solve this problem. However, similar to other deep learning methods, the instance segmentation requires a large scale training dataset and high quality annotation in order to achieve a considerable performance. In practice, preparing the training dataset is a challenge since annotators have to deal with a large image, e.g 2K resolution, and extremely dense objects in this problem. In this work, we present a novel method to tackle the problem of missing annotation in instance segmentation in 2D quantum material identification. We propose a new mechanism for automatically detecting false negative objects and an attention based loss strategy to reduce the negative impact of these objects contributing to the overall loss function. We experiment on the 2D material detection datasets, and the experiments show our method outperforms previous works.
Nguyen XB, Bisht A, Churchill H, Luu K. Two-dimensional quantum material identification via self-attention and soft-labeling in deep learning. arXiv preprint arXiv:2205.15948. 2022 May 31.
@article{nguyen2022two, title={Two-dimensional quantum material identification via self-attention and soft-labeling in deep learning}, author={Nguyen, Xuan Bac and Bisht, Apoorva and Churchill, Hugh and Luu, Khoa}, journal={arXiv preprint arXiv:2205.15948}, year={2022} }
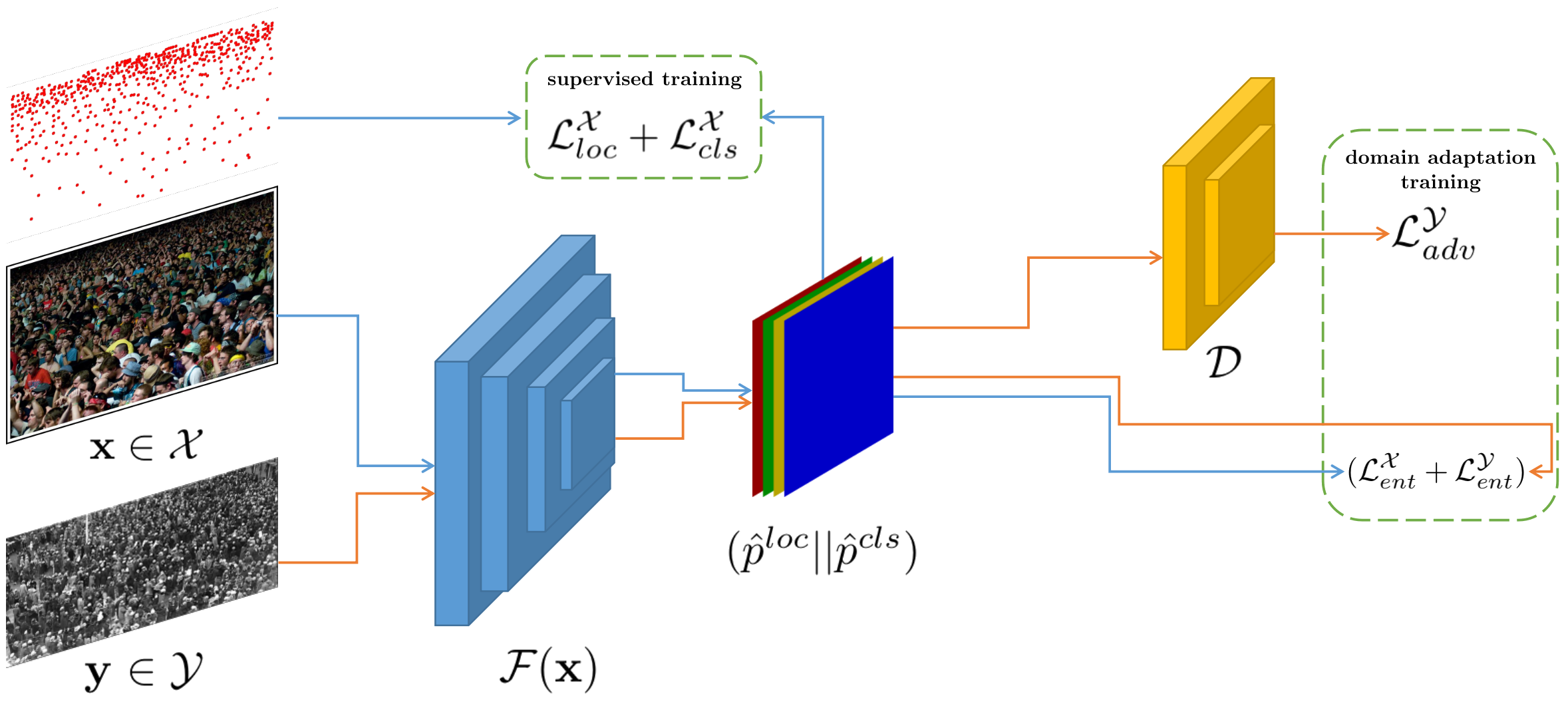
Self-supervised Domain Adaptation in Crowd Counting
Project Page | Paper
Self-training crowd counting has not been attentively
explored though it is one of the important challenges in
computer vision. In practice, the fully supervised
methods usually require an intensive resource of manual
annotation. In order to address this challenge, this
work introduces a new approach to utilize existing
datasets with ground truth to produce more robust
predictions on unlabeled datasets, named domain
adaptation, in crowd counting. While the network is
trained with labeled data, samples without labels from
the target domain are also added to the training
process. In this process, the entropy map is computed
and minimized in addition to the adversarial training
process designed in parallel.
Experiments on
Shanghaitech, UCF_CC_50, and UCF-QNRF datasets prove a
more generalized improvement of our method over the
other state-of-the-arts in the cross-domain setting.
Nguyen, P., Quach, K. G., Duong, C. N., Phung, S. L., Le, N., & Luu, K. (2022). Multi-Camera Multi-Object Tracking on the Move via Single-Stage Global Association Approach. arXiv preprint arXiv:2211.09663.
@article{nguyen2022multi, title={Multi-Camera Multi-Object Tracking on the Move via Single-Stage Global Association Approach}, author={Nguyen, Pha and Quach, Kha Gia and Duong, Chi Nhan and Phung, Son Lam and Le, Ngan and Luu, Khoa}, journal={arXiv preprint arXiv:2211.09663}, year={2022} }
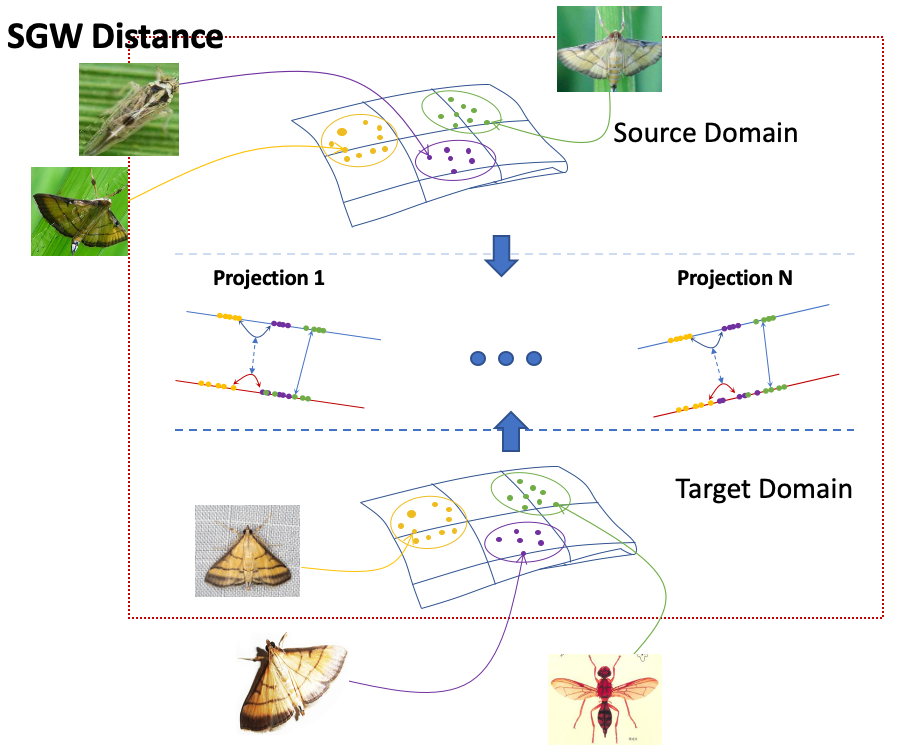
OTAdapt: Optimal Transport-based Approach For Unsupervised Domain Adaptation
Paper
Unsupervised domain adaptation is one of the challenging problems in computer vision. This paper presents a novel approach to unsupervised domain adaptations based on the optimal transport-based distance. Our approach allows aligning target and source domains without the requirement of meaningful metrics across domains. In addition, the proposal can associate the correct mapping between source and target domains and guarantee a constraint of topology between source and target domains. The proposed method is evaluated on different datasets in various problems, i.e. (i) digit recognition on MNIST, MNISTM, USPS datasets, (ii) Object recognition on Amazon, Webcam, DSLR, and VisDA datasets, (iii) Insect Recognition on the IP102 dataset. The experimental results show our proposed method consistently improves performance accuracy. Also, our framework can be incorporated with any other CNN frameworks within an end-to-end deep network design for recognition problems to improve their performance.
T. -D. Truong, R. T. N. Chappa, X. -B. Nguyen, N. Le, A. P. G. Dowling and K. Luu, "OTAdapt: Optimal Transport-based Approach For Unsupervised Domain Adaptation," 2022 26th International Conference on Pattern Recognition (ICPR), Montreal, QC, Canada, 2022, pp. 2850-2856, doi: 10.1109/ICPR56361.2022.9956335.
@article{truong2022otadapt, author={Truong, Thanh-Dat and Chappa, Ravi Teja Nvs and Nguyen, Xuan-Bac and Le, Ngan and Dowling, Ashley P.G. and Luu, Khoa}, journal={2022 26th International Conference on Pattern Recognition (ICPR)}, title={OTAdapt: Optimal Transport-based Approach For Unsupervised Domain Adaptation}, year={2022}, pages={2850-2856}, doi={10.1109/ICPR56361.2022.9956335}}
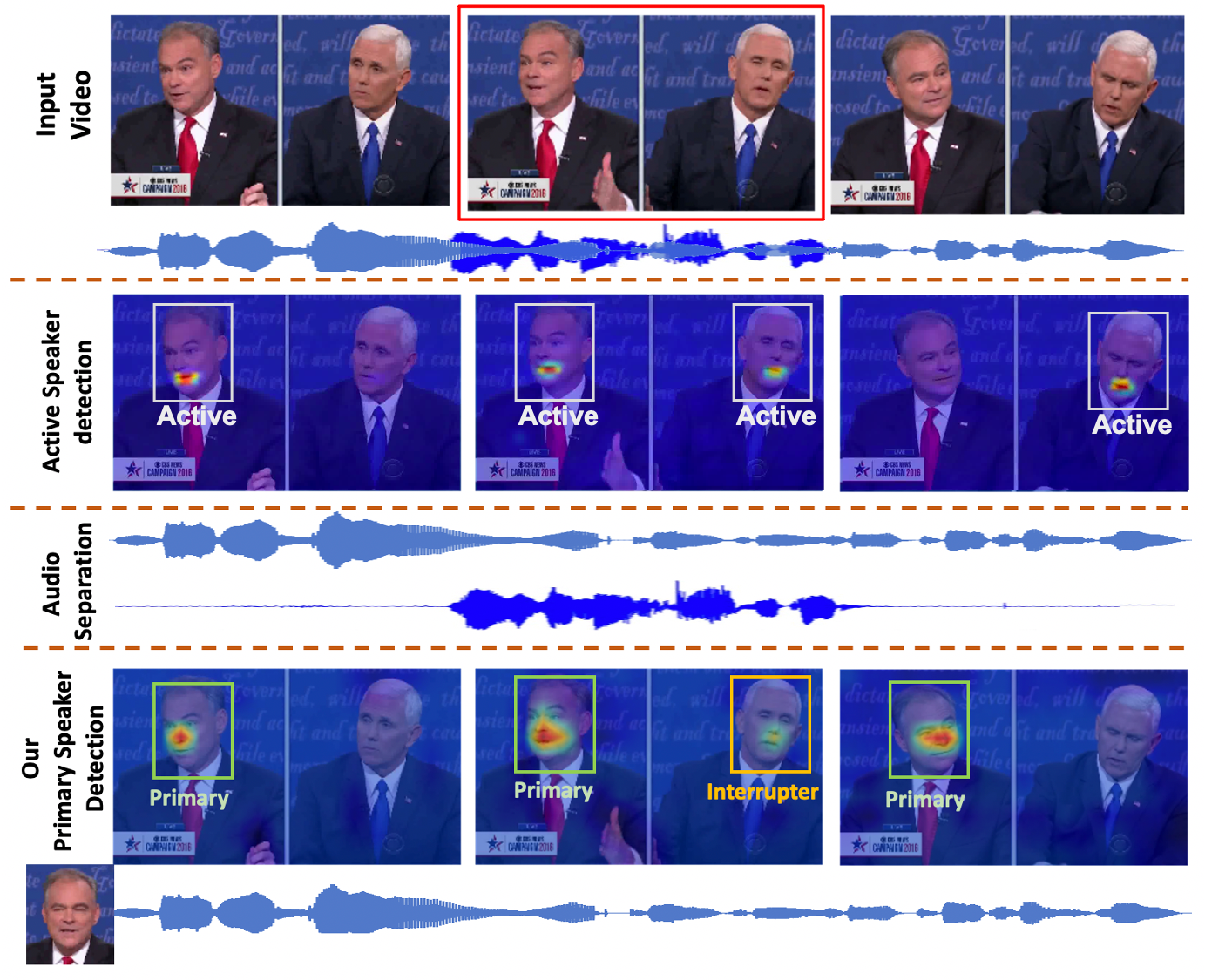
The Right to Talk: An audio-visual Transformer Approach
Turn-taking has played an essential role in structuring the regulation of a conversation. The task of identifying the main speaker (who is properly taking his/her turn of speaking) and the interrupters (who are interrupting or reacting to the main speaker's utterances) remains a challenging task. Although some prior methods have partially addressed this task, there still remain some limitations. Firstly, a direct association of Audio and Visual features may limit the correlations to be extracted due to different modalities. Secondly, the relationship across temporal segments helping to maintain the consistency of localization, separation and conversation contexts is not effectively exploited. Finally, the interactions between speakers that usually contain the tracking and anticipatory decisions about transition to a new speaker is usually ignored. Therefore, this work introduces a new Audio-Visual Transformer approach to the problem of localization and highlighting the main speaker in both audio and visual channels of a multi-speaker conversation video in the wild. The proposed method exploits different types of correlations presented in both visual and audio signals. The temporal audio-visual relationships across spatial-temporal space are anticipated and optimized via the self-attention mechanism in a Transformer structure. Moreover, a newly collected dataset is introduced for the main speaker detection. To the best of our knowledge, it is one of the first studies that is able to automatically localize and highlight the main speaker in both visual and audio channels in multi-speaker conversation videos.
Truong, Thanh-Dat, Chi Nhan Duong, Hoang Anh Pham, Bhiksha Raj, Ngan Le, and Khoa Luu. "The right to talk: An audio-visual transformer approach." In Proceedings of the IEEE/CVF International Conference on Computer Vision, pp. 1105-1114. 2021.
@InProceedings{truong2021right2talk, author = {Truong, Thanh-Dat and Duong, Chi Nhan and De Vu, The and Pham, Hoang Anh and Raj, Bhiksha and Le, Ngan and Luu, Khoa}, title = {The Right To Talk: An Audio-Visual Transformer Approach}, booktitle = {Proceedings of the IEEE/CVF International Conference on Computer Vision (ICCV)}, month = {October}, year = {2021}, pages = {1105-1114} }
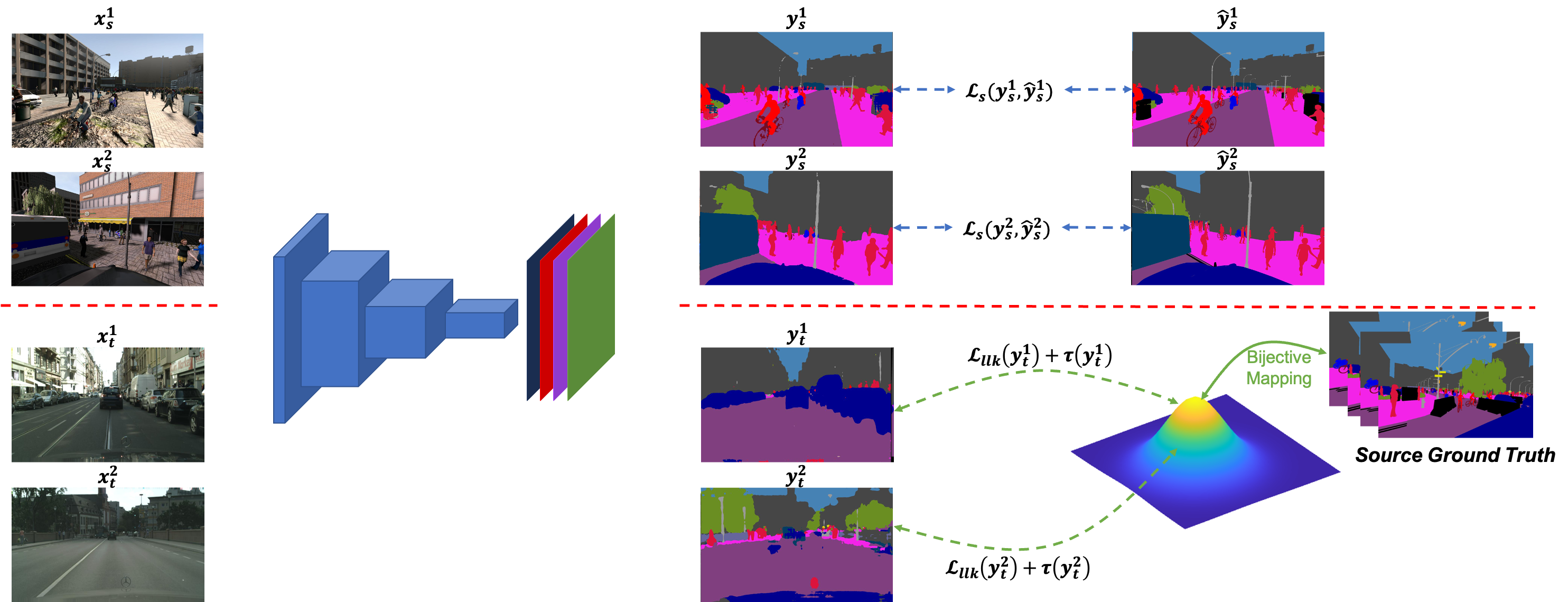
BiMal: Bijective Maximum Likelihood Approach to Domain Adaptation in Semantic Scene Segmentation
Semantic segmentation aims to predict pixel-level labels. It has become a popular task in various computer vision applications. While fully supervised segmentation methods have achieved high accuracy on large-scale vision datasets, they are unable to generalize on a new test environment or a new domain well. In this work, we first introduce a new Un- aligned Domain Score to measure the efficiency of a learned model on a new target domain in unsupervised manner. Then, we present the new Bijective Maximum Likelihood (BiMaL) loss that is a generalized form of the Adversarial Entropy Minimization without any assumption about pixel independence. We have evaluated the proposed BiMaL on two domains. The proposed BiMaL approach consistently outperforms the SOTA methods on empirical experiments on "SYNTHIA to Cityscapes", "GTA5 to Cityscapes", and "SYNTHIA to Vistas".
Thanh-Dat Truong, Chi Nhan Duong, Ngan Le, Son Lam Phung, Chase Rainwater, Khoa Luu.BiMal: Bijective Maximum Likelihood Approach to Domain Adaptation in Semantic Scene Segmentation. Proceedings of the IEEE/CVF International Conference on Computer Vision (ICCV), 2021, pp. 8548-8557
@InProceedings{truong2021bimal, author = {Truong, Thanh-Dat and Duong, Chi Nhan and Le, Ngan and Phung, Son Lam and Rainwater, Chase and Luu, Khoa}, title = {BiMaL: Bijective Maximum Likelihood Approach to Domain Adaptation in Semantic Scene Segmentation}, booktitle = {Proceedings of the IEEE/CVF International Conference on Computer Vision (ICCV)}, month = {October}, year = {2021}, pages = {8548-8557} }
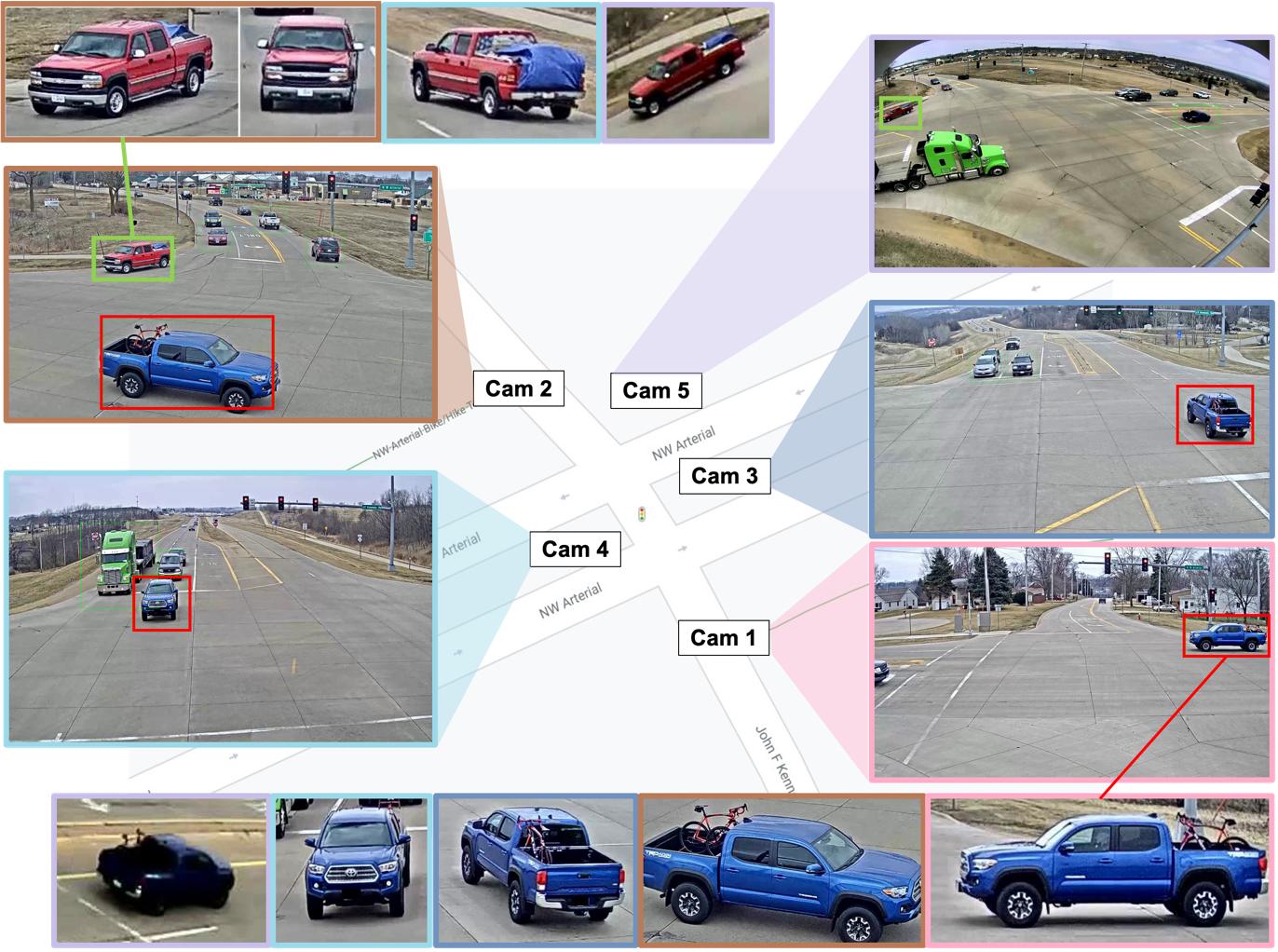
DyGLIP: A Dynamic Graph Model with Link Prediction for Accurate Multi-Camera Multiple Object Tracking
Paper | Poster | Video | Code
Multi-Camera Multiple Object Tracking (MC-MOT) is a significant computer vision problem due to its emerging applicability in several real-world applications. Despite a large number of existing works, solving the data association problem in any MC-MOT pipeline is arguably one of the most challenging tasks. Developing a robust MC-MOT system, however, is still highly challenging due to many practical issues such as inconsistent lighting conditions, varying object movement patterns, or the trajectory occlusions of the objects between the cameras. To address these problems, this work, therefore, proposes a new Dynamic Graph Model with Link Prediction (DyGLIP) approach to solve the data association task. Compared to existing methods, our new model offers several advantages, including better feature representations and the ability to recover from lost tracks during camera transitions. Moreover, our model works gracefully regardless of the overlapping ratios between the cameras. Experimental results show that we outperform existing MC-MOT algorithms by a large margin on several practical datasets. Notably, our model works favorably on online settings but can be extended to an incremental approach for large-scale datasets.
Quach, Kha Gia, Pha Nguyen, Huu Le, Thanh-Dat Truong, Chi Nhan Duong, Minh-Triet Tran, and Khoa Luu. "Dyglip: A dynamic graph model with link prediction for accurate multi-camera multiple object tracking." In Proceedings of the IEEE/CVF Conference on Computer Vision and Pattern Recognition, pp. 13784-13793. 2021.
@inproceedings{quach2021dyglip, title={Dyglip: A dynamic graph model with link prediction for accurate multi-camera multiple object tracking}, author={Quach, Kha Gia and Nguyen, Pha and Le, Huu and Truong, Thanh-Dat and Duong, Chi Nhan and Tran, Minh-Triet and Luu, Khoa}, booktitle={Proceedings of the IEEE/CVF Conference on Computer Vision and Pattern Recognition}, pages={13784--13793}, year={2021} }
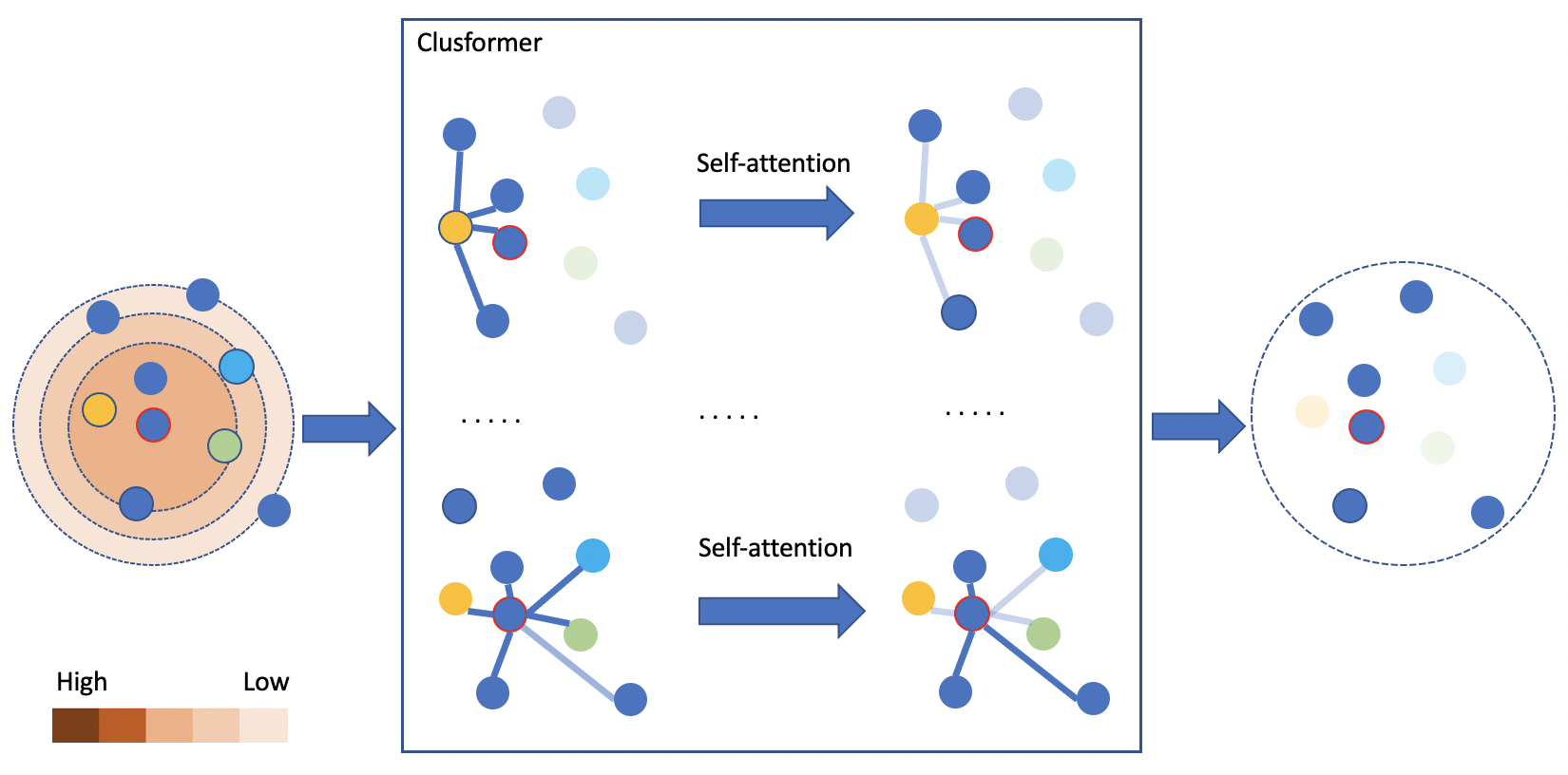
Clusformer: A transformer based clustering approach to unsupervised large-scale face and visual landmark recognition
Paper
The research in automatic unsupervised visual clustering has received considerable attention over the last couple years. It aims at explaining distributions of unlabeled visual images by clustering them via a parameterized model of appearance. Graph Convolutional Neural Networks (GCN) have recently been one of the most popular clustering methods. However, it has reached some limitations. Firstly, it is quite sensitive to hard or noisy samples. Secondly, it is hard to investigate with various deep network models due to its computational training time. Finally, it is hard to design an end-to-end training model between the deep feature extraction and GCN clustering modeling. This work therefore presents the Clusformer, a simple but new perspective of Transformer based approach, to automatic visual clustering via its unsupervised attention mechanism. The proposed method is able to robustly deal with noisy or hard samples. It is also flexible and effective to collaborate with different deep network models with various model sizes in an end-to-end framework. The proposed method is evaluated on two popular large-scale visual databases, ie Google Landmark and MS-Celeb-1M face database, and outperforms prior unsupervised clustering methods. Code will be available at https://github. com/VinAIResearch/Clusformer
Nguyen XB, Bui DT, Duong CN, Bui TD, Luu K. Clusformer: A Transformer based Clustering Approach to Unsupervised Large-scale Face and Visual Landmark Recognition. In2021 IEEE/CVF Conference on Computer Vision and Pattern Recognition (CVPR) 2021 Jun 1 (pp. 10842-10851). IEEE Computer Society.
@InProceedings{Nguyen_2021_CVPR, author = {Nguyen, Xuan-Bac and Bui, Duc Toan and Duong, Chi Nhan and Bui, Tien D. and Luu, Khoa}, title = {Clusformer: A Transformer Based Clustering Approach to Unsupervised Large-Scale Face and Visual Landmark Recognition}, booktitle = {Proceedings of the IEEE/CVF Conference on Computer Vision and Pattern Recognition (CVPR)}, month = {June}, year = {2021}, pages = {10847-10856} }
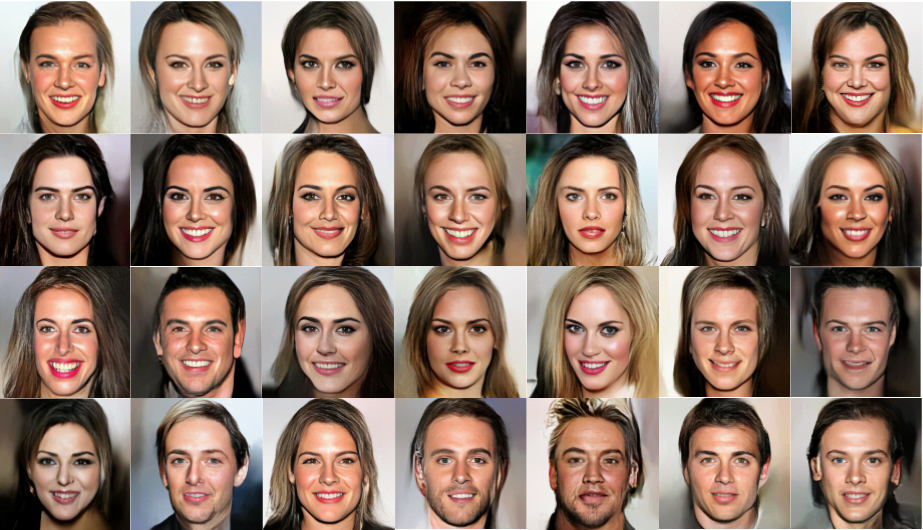
Fast Flow Reconstruction via Robust Invertible NxN Convolution
Paper
Flow-based generative models have recently become one of the most efficient approaches to model data generation. Indeed, they are constructed with a sequence of invertible and tractable transformations. Glow first introduced a simple type of generative flow using an invertible 1×1 convolution. However, the 1×1 convolution suffers from limited flexibility compared to the standard convolutions. In this paper, we propose a novel invertible n×n convolution approach that overcomes the limitations of the invertible 1×1 convolution. In addition, our proposed network is not only tractable and invertible but also uses fewer parameters than standard convolutions. The experiments on CIFAR-10, ImageNet and Celeb-HQ datasets, have shown that our invertible n×n convolution helps to improve the performance of generative models significantly.
Truong, Thanh-Dat, Chi Nhan Duong, Minh-Triet Tran, Ngan Le, and Khoa Luu. "Fast flow reconstruction via robust invertible n× n convolution." Future Internet 13, no. 7 (2021): 179.
@article{truong2021fast, title={Fast flow reconstruction via robust invertible n$\times$ n convolution}, author={Truong, Thanh-Dat and Duong, Chi Nhan and Tran, Minh-Triet and Le, Ngan and Luu, Khoa}, journal={Future Internet}, volume={13}, number={7}, pages={179}, year={2021}, publisher={MDPI} }
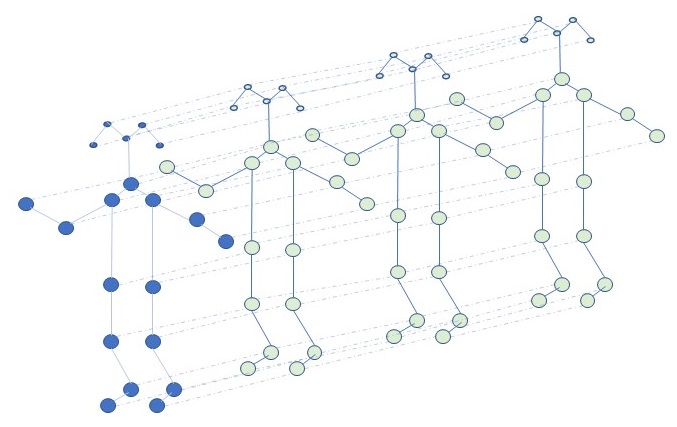
Movement Analysis for Neurological and Musculoskeletal Disorders Using Graph Convolutional Neural Network
Paper
Using optical motion capture and wearable sensors is a common way to analyze impaired movement in individuals with neurological and musculoskeletal disorders. However, using optical motion sensors and wearable sensors is expensive and often requires highly trained professionals to identify specific impairments. In this work, we proposed a graph convolutional neural network that mimics the intuition of physical therapists to identify patient-specific impairments based on video of a patient. In addition, two modeling approaches are compared: a graph convolutional network applied solely on skeleton input data and a graph convolutional network accompanied with a 1-dimensional convolutional neural network (1D-CNN). Experiments on the dataset showed that the proposed method not only improves the correlation of the predicted gait measure with the ground truth value (speed = 0.791, gait deviation index (GDI) = 0.792) but also enables faster training with fewer parameters. In conclusion, the proposed method shows that the possibility of using video-based data to treat neurological and musculoskeletal disorders with acceptable accuracy instead of depending on the expensive and labor-intensive optical motion capture systems.
Jalata, Ibsa K., Thanh-Dat Truong, Jessica L. Allen, Han-Seok Seo, and Khoa Luu. "Movement analysis for neurological and musculoskeletal disorders using graph convolutional neural network." Future Internet 13, no. 8 (2021): 194.
@article{jalata2021movement, title={Movement analysis for neurological and musculoskeletal disorders using graph convolutional neural network}, author={Jalata, Ibsa K and Truong, Thanh-Dat and Allen, Jessica L and Seo, Han-Seok and Luu, Khoa}, journal={Future Internet}, volume={13}, number={8}, pages={194}, year={2021}, publisher={MDPI} }
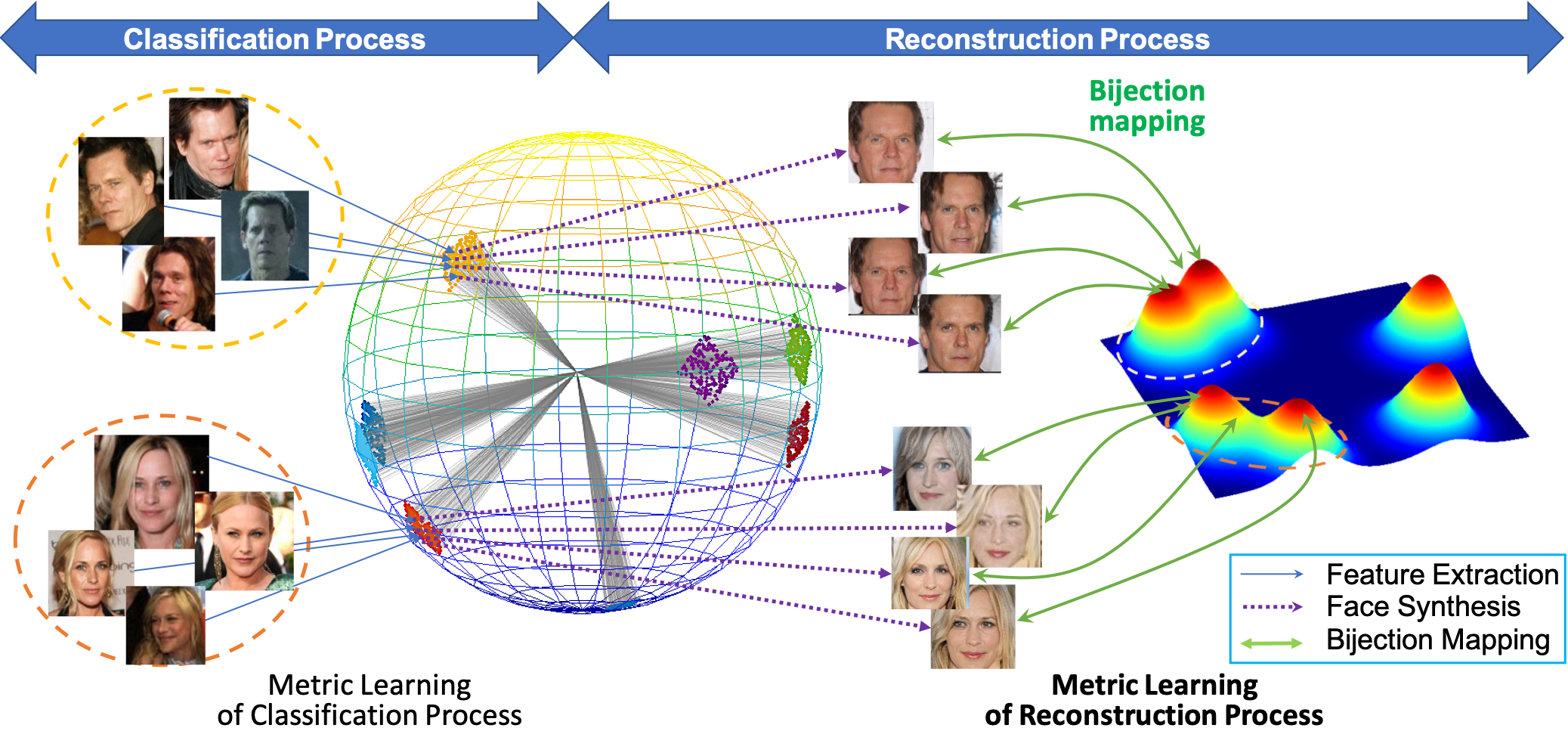
Vec2Face: Unveil Human Faces from their Blackbox Features in Face Recognition
Paper Video |
Unveiling face images of a subject given his/her high-level representations extracted from a blackbox Face Recognition engine is extremely challenging. It is because the limitations of accessible information from that engine including its structure and uninterpretable extracted features. This paper presents a novel generative structure with Bijective Metric Learning, namely Bijective Generative Adversarial Networks in a Distillation framework (DiBiGAN), for synthesizing faces of an identity given that person's features. In order to effectively address this problem, this work firstly introduces a bijective metric so that the distance measurement and metric learning process can be directly adopted in image domain for an image reconstruction task. Secondly, a distillation process is introduced to maximize the information exploited from the blackbox face recognition engine. Then a Feature-Conditional Generator Structure with Exponential Weighting Strategy is presented for a more robust generator that can synthesize realistic faces with ID preservation. Results on several benchmarking datasets including CelebA, LFW, AgeDB, CFP-FP against matching engines have demonstrated the effectiveness of DiBiGAN on both image realism and ID preservation properties.
Chi Nhan Duong, Thanh-Dat Truong, Khoa Luu, Kha Gia Quach, Hung Bui, Kaushik Roy. Vec2Face: Unveil Human Faces from their Blackbox Features in Face Recognition. Proceedings of the IEEE/CVF Conference on Computer Vision and Pattern Recognition (CVPR), 2020, pp. 6132-6141
@InProceedings{duong2020vec2face, author = {Duong, Chi Nhan and Truong, Thanh-Dat and Luu, Khoa and Quach, Kha Gia and Bui, Hung and Roy, Kaushik}, title = {Vec2Face: Unveil Human Faces From Their Blackbox Features in Face Recognition}, booktitle = {Proceedings of the IEEE/CVF Conference on Computer Vision and Pattern Recognition (CVPR)}, month = {June}, year = {2020} }
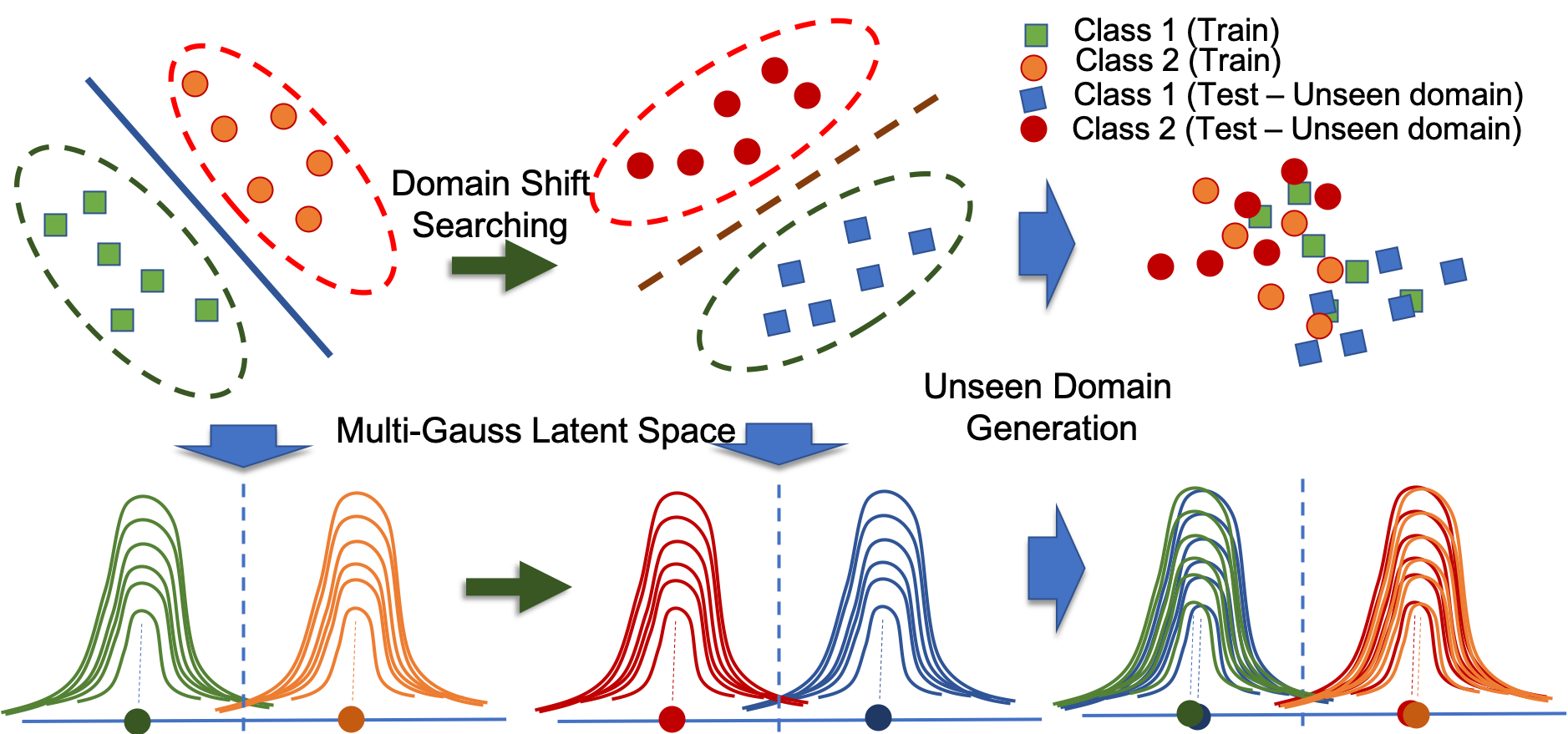
Domain Generalization via Universal Non-volume Preserving Approach
Paper
Recognition across domains has recently become an active topic in the research community. However, it has been largely overlooked in the problem of recognition in new unseen domains. Under this condition, the delivered deep network models are unable to be updated, adapted, or fine-tuned. Therefore, recent deep learning techniques, such as domain adaptation, feature transferring, and fine-tuning, cannot be applied. This paper presents a novel approach to the problem of domain generalization in the context of deep learning. The proposed method 1 is evaluated on different datasets in various problems, i.e. (i) digit recognition on MNIST, SVHN, and MNIST-M, (ii) face recognition on Extended Yale-B, CMU-PIE and CMU-MPIE, and (iii) pedestrian recognition on RGB and Thermal image datasets. The experimental results show that our proposed method consistently improves performance accuracy. It can also be easily incorporated with any other CNN frameworks within an end-to-end deep network design for object detection and recognition problems to improve their performance.
D. T. Truong, C. Nhan Duong, K. Luu, M. -T. Tran and N. Le, "Domain Generalization via Universal Non-volume Preserving Approach," 2020 17th Conference on Computer and Robot Vision (CRV), Ottawa, ON, Canada, 2020, pp. 93-100, doi: 10.1109/CRV50864.2020.00021.
@INPROCEEDINGS{truong2020unvp, author={Truong, Dat T. and Nhan Duong, Chi and Luu, Khoa and Tran, Minh-Triet and Le, Ngan}, booktitle={2020 17th Conference on Computer and Robot Vision (CRV)}, title={Domain Generalization via Universal Non-volume Preserving Approach}, year={2020}, volume={}, number={}, pages={93-100}, doi={10.1109/CRV50864.2020.00021}}
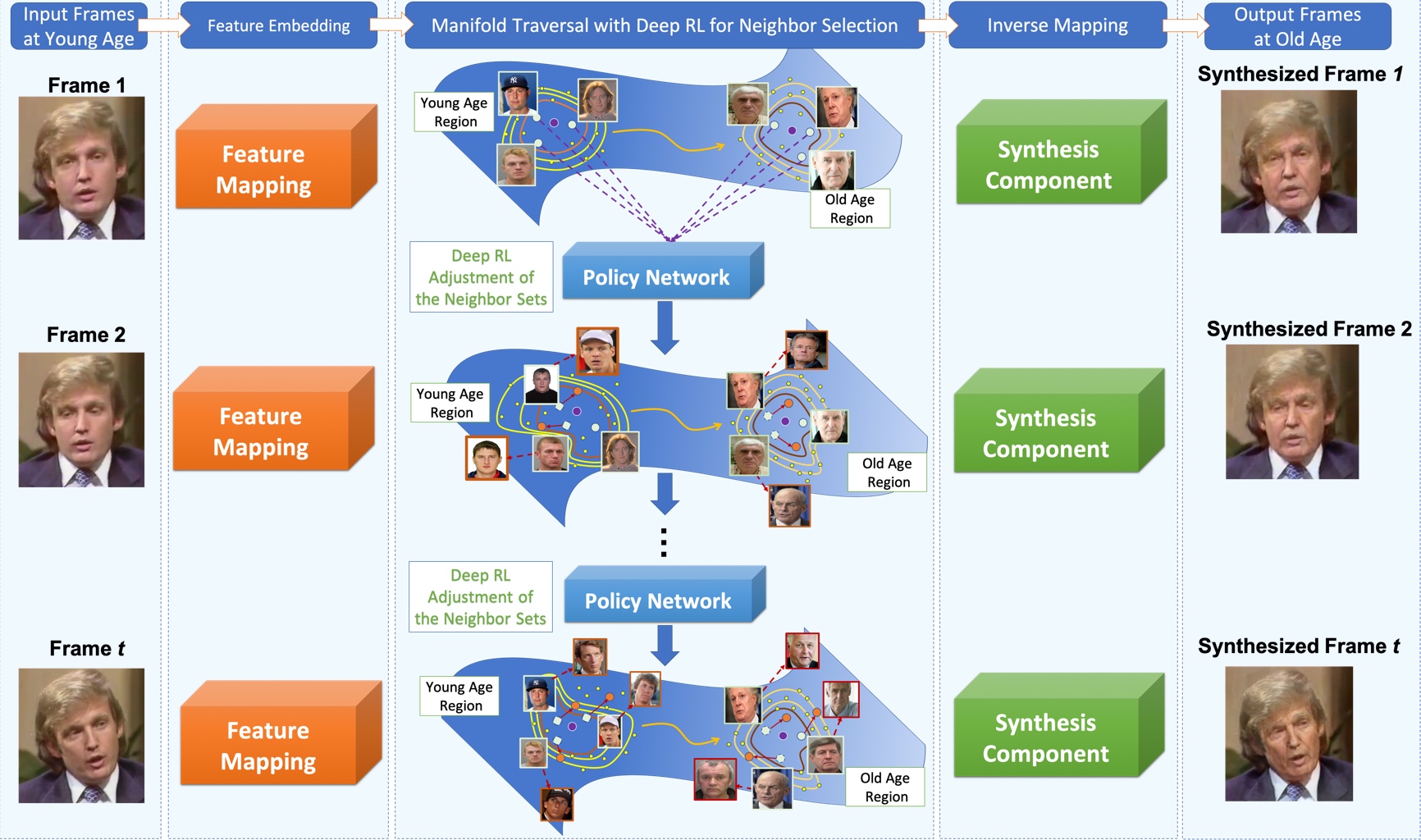
Automatic Face Aging in Videos via Deep Reinforcement Learning
Paper
This paper presents a novel approach for synthesizing automatically age-progressed facial images in video sequences using Deep Reinforcement Learning. The proposed method models facial structures and the longitudinal face-aging process of given subjects coherently across video frames. The approach is optimized using a long-term reward, Reinforcement Learning function with deep feature extraction from Deep Convolutional Neural Network. Unlike previous age-progression methods that are only able to synthesize an aged likeness of a face from a single input image, the proposed approach is capable of age-progressing facial likenesses in videos with consistently synthesized facial features across frames. In addition, the deep reinforcement learning method guarantees preservation of the visual identity of input faces after age-progression. Results on videos of our new collected aging face AGFW-v2 database demonstrate the advantages of the proposed solution in terms of both quality of age-progressed faces, temporal smoothness, and cross-age face verification.
Duong, Chi Nhan, Khoa Luu, Kha Gia Quach, Nghia Nguyen, Eric Patterson, Tien D. Bui, and Ngan Le. "Automatic face aging in videos via deep reinforcement learning." In Proceedings of the IEEE/CVF Conference on Computer Vision and Pattern Recognition, pp. 10013-10022. 2019.
@INPROCEEDINGS{duong2019automatic, author = {Automatic Face Aging in Videos via Deep Reinforcement Learning}, title = {Chi Nhan Duong and Khoa Luu and Kha Gia Quach and Nghia Nguyen and Eric Patterson and Tien D. Bui and Ngan Le}, booktitle={IEEE Conference on Computer Vision and Pattern Recognition (CVPR)}, year={2019} }
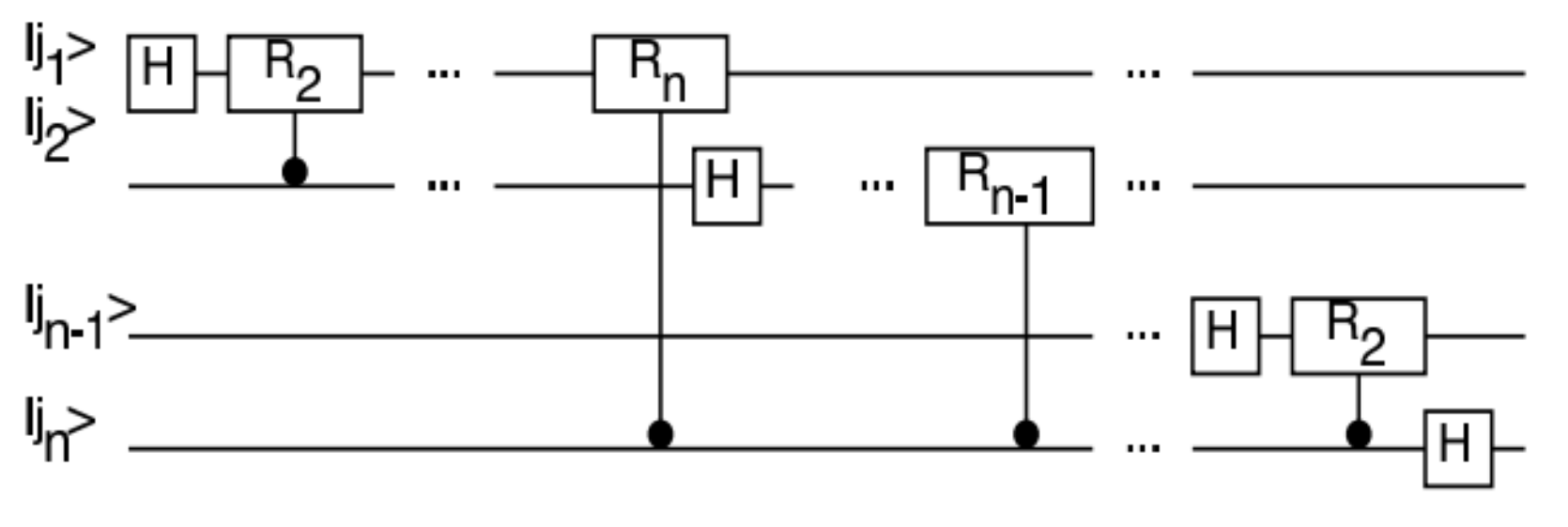
Image Processing in Quantum Computers
Paper
Quantum Image Processing (QIP)is an exciting new field showing a lot of promise as a powerful addition to the arsenal of Image Processing techniques. Representing image pixel by pixel using classical information requires an enormous amount of computational resources. Hence, exploring methods to represent images in a different paradigm of information is important. In this work, we study the representation of images in Quantum Information. The main motivation for this pursuit is the ability of storing N bits of classical information in only log(2N) quantum bits (qubits). The promising first step was the exponentially efficient implementation of the Fourier transform in quantum computers as compared to Fast Fourier Transform in classical computers. In addition, images encoded in quantum information could obey unique quantum properties like superposition or entanglement.
Dendukuri, Aditya, and Khoa Luu. "Image processing in quantum computers." In Proceedings of Quantum Techniques in Machine Learning. 2019.
@article{dendukuri2018image, title={Image processing in quantum computers}, author={Dendukuri, Aditya and Luu, Khoa}, journal={Proceedings of Quantum Techniques in Machine Learning}, year={2019} }